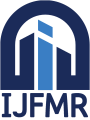
International Journal For Multidisciplinary Research
E-ISSN: 2582-2160
•
Impact Factor: 9.24
A Widely Indexed Open Access Peer Reviewed Multidisciplinary Bi-monthly Scholarly International Journal
Home
Research Paper
Submit Research Paper
Publication Guidelines
Publication Charges
Upload Documents
Track Status / Pay Fees / Download Publication Certi.
Editors & Reviewers
View All
Join as a Reviewer
Get Membership Certificate
Current Issue
Publication Archive
Conference
Publishing Conf. with IJFMR
Upcoming Conference(s) ↓
WSMCDD-2025
GSMCDD-2025
Conferences Published ↓
RBS:RH-COVID-19 (2023)
ICMRS'23
PIPRDA-2023
Contact Us
Plagiarism is checked by the leading plagiarism checker
Call for Paper
Volume 7 Issue 2
March-April 2025
Indexing Partners






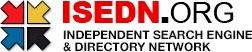

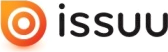


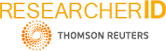
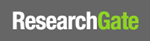
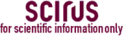
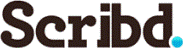

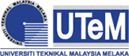
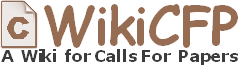
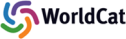
The Detection and Classification of Diabetic Retinopathy using the Architectures of Deep Learning
Author(s) | Vandana, Vijay laxmi |
---|---|
Country | India |
Abstract | Abstract: Diabetic retinopathy is a severe problem of long-term diabetes, which affects the human eye and gradually leading to permanent blindness. The early detection of DR is crucial for effective treatment, as symptoms often manifest in later stages. The manual grading of retinal images is time-consuming, prone to errors, and lacks patient-friendliness. In this study, we propose two deep learning (DL) architectures, a hybrid network combining VGG16 and XGBoost Classi- fier, and the DenseNet 121 network, for Diabetic retionopathy detection and classification. To evaluate the two DL models, we preprocessed a collection of retinal images obtained from the APTOS 2019 Blindness Detection Kaggle Dataset. This dataset exhibits not balanced image class distribution, which we addressed through appropriate balancing techniques. The performance of the considered models was assessed in terms of accuracy. The results showed that the hybrid network achieved an accuracy of 79.50%, while the DenseNet 121 model achieved an accuracy of 97.30%. Furthermore, a comparative analysis with existing methods utilizing the same dataset revealed the superior performance of the DenseNet 121 network. The findings of this study demonstrate the potential of DL architectures for the early detection and classification of DR. The better performance of the DenseNet 121 model highlights its effectiveness in this domain. The implementation of such automated methods can sig- nificantly improve the efficiency and accuracy of DR diagnosis, benefiting both healthcare providers and patients. |
Keywords | CNN; Diabetic retinopathy; data balance; XGBoost ; DenseNet . |
Field | Computer > Artificial Intelligence / Simulation / Virtual Reality |
Published In | Volume 5, Issue 6, November-December 2023 |
Published On | 2023-12-25 |
DOI | https://doi.org/10.36948/ijfmr.2023.v05i06.10837 |
Short DOI | https://doi.org/gs98sq |
Share this

E-ISSN 2582-2160

CrossRef DOI is assigned to each research paper published in our journal.
IJFMR DOI prefix is
10.36948/ijfmr
Downloads
All research papers published on this website are licensed under Creative Commons Attribution-ShareAlike 4.0 International License, and all rights belong to their respective authors/researchers.
