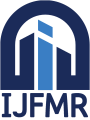
International Journal For Multidisciplinary Research
E-ISSN: 2582-2160
•
Impact Factor: 9.24
A Widely Indexed Open Access Peer Reviewed Multidisciplinary Bi-monthly Scholarly International Journal
Home
Research Paper
Submit Research Paper
Publication Guidelines
Publication Charges
Upload Documents
Track Status / Pay Fees / Download Publication Certi.
Editors & Reviewers
View All
Join as a Reviewer
Reviewer Referral Program
Get Membership Certificate
Current Issue
Publication Archive
Conference
Publishing Conf. with IJFMR
Upcoming Conference(s) ↓
WSMCDD-2025
Conferences Published ↓
RBS:RH-COVID-19 (2023)
ICMRS'23
PIPRDA-2023
Contact Us
Plagiarism is checked by the leading plagiarism checker
Call for Paper
Volume 6 Issue 4
July-August 2024
Indexing Partners






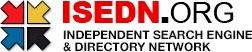

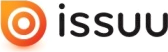


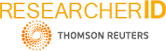
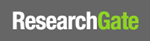
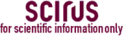
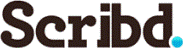

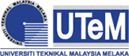
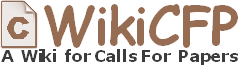
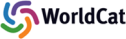
Wart Classification Using Logistic Regression: Analysis Based on Data Partitioning, Error Rate Comparison and Feature Selection
Author(s) | Siddharth Kumar |
---|---|
Country | India |
Abstract | Accurate classification of cancerous warts is pivotal for effective medical intervention, and logistic regression serves as a promising tool for this purpose. This study delves into the realm of wart classification using logistic regression, with a specific focus on three key aspects: data partitioning, error rate comparison, and feature selection. Logistic regression demonstrates commendable accuracy during training, but an observed disparity between training and testing accuracy prompts a critical examination of potential overfitting. Data partitioning unveils mixed results, enhancing overall testing accuracy while diminishing performance on partitioned datasets, emphasizing the importance of meticulous dataset splitting. Furthermore, the impact of feature selection on the model's performance is explored, underscoring the need for a detailed analysis of influential features. The study concludes by proposing future work, including addressing overfitting through regularization, investigating feature importance, exploring alternative classification algorithms, optimizing accuracy through ensemble methods, and expanding the dataset for enhanced generalization. This research contributes to the advancement of wart classification methodologies, providing insights into logistic regression's application and paving the way for refined diagnostic tools in dermatological practice. |
Keywords | Warts, Logistic Regression, Malignant, Benign, Cryotherapy |
Field | Computer > Data / Information |
Published In | Volume 5, Issue 6, November-December 2023 |
Published On | 2023-12-30 |
Cite This | Wart Classification Using Logistic Regression: Analysis Based on Data Partitioning, Error Rate Comparison and Feature Selection - Siddharth Kumar - IJFMR Volume 5, Issue 6, November-December 2023. DOI 10.36948/ijfmr.2023.v05i06.11374 |
DOI | https://doi.org/10.36948/ijfmr.2023.v05i06.11374 |
Short DOI | https://doi.org/gtbtdw |
Share this
E-ISSN 2582-2160

CrossRef DOI is assigned to each research paper published in our journal.
IJFMR DOI prefix is
10.36948/ijfmr
Downloads
All research papers published on this website are licensed under Creative Commons Attribution-ShareAlike 4.0 International License, and all rights belong to their respective authors/researchers.
