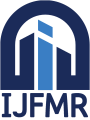
International Journal For Multidisciplinary Research
E-ISSN: 2582-2160
•
Impact Factor: 9.24
A Widely Indexed Open Access Peer Reviewed Multidisciplinary Bi-monthly Scholarly International Journal
Home
Research Paper
Submit Research Paper
Publication Guidelines
Publication Charges
Upload Documents
Track Status / Pay Fees / Download Publication Certi.
Editors & Reviewers
View All
Join as a Reviewer
Reviewer Referral Program
Get Membership Certificate
Current Issue
Publication Archive
Conference
Publishing Conf. with IJFMR
Upcoming Conference(s) ↓
WSMCDD-2025
Conferences Published ↓
RBS:RH-COVID-19 (2023)
ICMRS'23
PIPRDA-2023
Contact Us
Plagiarism is checked by the leading plagiarism checker
Call for Paper
Volume 6 Issue 4
July-August 2024
Indexing Partners






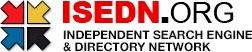

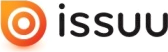


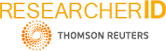
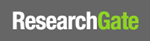
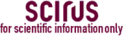
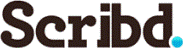

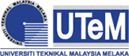
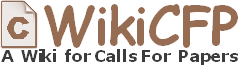
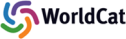
An Optimal Random Forest Model for Enhancing Decision-Making in Improving Students’ Performance via Educational Data Mining
Author(s) | Samuel Odoom, Eric Opoku Osei |
---|---|
Country | Ghana |
Abstract | The need for measures to enhance students’ performance, especially in Science and Math as a panacea for development in this 4th industrial revolution has become a global call. The large stream and heterogeneous nature of educational data make it difficult to use traditional statistical methods for data analysis to support decisions. However, Educational data mining has been effective and efficient in addressing this issue but much focus of researchers has been on only students' academic records to determine performance. This study sought to propose a Random Forest model to predict and enhance students’ performance. The study adopted a 5-stage mining process to mine psycho-socio-economic demographics educational data with Mutual information, Chi-squared test, Featurewiz, RandomizedSearch CV, and GridSearch CV to optimize the model’s performance. The study’s outcome revealed the key factors affecting students’ performance and that the model was enhanced by a 10.4% increment in precision and f1-score and 9.1% recall value, 7.1secs (62%) improvement in execution time and 78.7% improvement in Root Mean Square Error. This outcome remains a contribution to guiding decision-making in the educational setting and a basis for further studies on model optimization. |
Keywords | Enhancing students’ performance, Decision support model, Educational data mining, Optimized Random Forest model, and Machine Learning |
Field | Computer > Data / Information |
Published In | Volume 6, Issue 1, January-February 2024 |
Published On | 2024-01-04 |
Cite This | An Optimal Random Forest Model for Enhancing Decision-Making in Improving Students’ Performance via Educational Data Mining - Samuel Odoom, Eric Opoku Osei - IJFMR Volume 6, Issue 1, January-February 2024. DOI 10.36948/ijfmr.2024.v06i01.11584 |
DOI | https://doi.org/10.36948/ijfmr.2024.v06i01.11584 |
Short DOI | https://doi.org/gtdscr |
Share this
E-ISSN 2582-2160

CrossRef DOI is assigned to each research paper published in our journal.
IJFMR DOI prefix is
10.36948/ijfmr
Downloads
All research papers published on this website are licensed under Creative Commons Attribution-ShareAlike 4.0 International License, and all rights belong to their respective authors/researchers.
