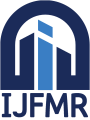
International Journal For Multidisciplinary Research
E-ISSN: 2582-2160
•
Impact Factor: 9.24
A Widely Indexed Open Access Peer Reviewed Multidisciplinary Bi-monthly Scholarly International Journal
Home
Research Paper
Submit Research Paper
Publication Guidelines
Publication Charges
Upload Documents
Track Status / Pay Fees / Download Publication Certi.
Editors & Reviewers
View All
Join as a Reviewer
Get Membership Certificate
Current Issue
Publication Archive
Conference
Publishing Conf. with IJFMR
Upcoming Conference(s) ↓
WSMCDD-2025
GSMCDD-2025
Conferences Published ↓
RBS:RH-COVID-19 (2023)
ICMRS'23
PIPRDA-2023
Contact Us
Plagiarism is checked by the leading plagiarism checker
Call for Paper
Volume 7 Issue 2
March-April 2025
Indexing Partners






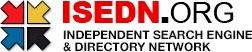

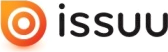


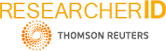
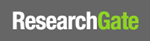
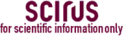
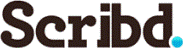

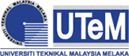
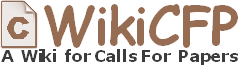
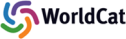
Deep Learning for Holistic Mental Illness Diagnosis with Multimodal Imaging
Author(s) | Vijay Kumar Ravi, Kavita Ravat |
---|---|
Country | India |
Abstract | Detecting and diagnosing mental illnesses is a complex task due to the heterogeneous nature of these conditions, which encompass various types and subtypes. Timely identification and diagnosis are essential for advancing mental health research, improving early intervention, and developing effective therapies. However, existing methods have primarily focused on categorizing and diagnosing single mental illness types, often prioritizing patient survival over comprehensive treatment. This research introduces a deep learning-based approach to establish a universal framework for the detection, diagnosis, symptom-based prediction, and screening of diverse mental illnesses, their subtypes, and associated factors using a diverse dataset comprising images and clinical scans. The proposed architecture leverages a VGG-19-based 3D-convolutional neural network for robust feature extraction and employs a random forest algorithm for regression tasks. To create a comprehensive dataset, we incorporate results from the DAIC-WOZ laboratory dataset, imaging studies, and biopsy reports, moving beyond sole reliance on clinical images. The initial step involves distinguishing between healthy and unhealthy depression, sleep disorder, Alzheimer's, schizophrenia, and epilepsy conditions and subsequently classifying depression, sleep disorder, Alzheimer's, schizophrenia, and epilepsy cases into their specific subtypes while assessing their severity and growth patterns. Our model is designed to predict risk levels at various time intervals, utilizing the available pertinent factors consistently across different diagnostic tools. Our results demonstrate promising accuracy, with a 98.80% success rate in classifying them into their respective subtypes, a sensitivity of 98.24%, and a specificity of 98.49%. This research represents a significant step toward a more holistic and precise approach to mental illness detection, diagnosis, and management, performing 4.74% better than other state-of-the-art AI models and offering potential benefits for individuals and mental health professionals alike. |
Keywords | Computer-aided Diagnosis; Electroencephalogram; Mental Illness; Recurrent Neural Network |
Field | Engineering |
Published In | Volume 6, Issue 1, January-February 2024 |
Published On | 2024-01-20 |
DOI | https://doi.org/10.36948/ijfmr.2024.v06i01.12148 |
Short DOI | https://doi.org/gtfmr4 |
Share this

E-ISSN 2582-2160

CrossRef DOI is assigned to each research paper published in our journal.
IJFMR DOI prefix is
10.36948/ijfmr
Downloads
All research papers published on this website are licensed under Creative Commons Attribution-ShareAlike 4.0 International License, and all rights belong to their respective authors/researchers.
