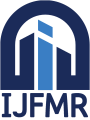
International Journal For Multidisciplinary Research
E-ISSN: 2582-2160
•
Impact Factor: 9.24
A Widely Indexed Open Access Peer Reviewed Multidisciplinary Bi-monthly Scholarly International Journal
Home
Research Paper
Submit Research Paper
Publication Guidelines
Publication Charges
Upload Documents
Track Status / Pay Fees / Download Publication Certi.
Editors & Reviewers
View All
Join as a Reviewer
Get Membership Certificate
Current Issue
Publication Archive
Conference
Publishing Conf. with IJFMR
Upcoming Conference(s) ↓
WSMCDD-2025
GSMCDD-2025
Conferences Published ↓
RBS:RH-COVID-19 (2023)
ICMRS'23
PIPRDA-2023
Contact Us
Plagiarism is checked by the leading plagiarism checker
Call for Paper
Volume 7 Issue 2
March-April 2025
Indexing Partners






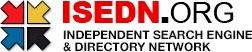

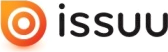


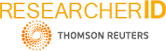
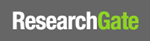
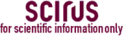
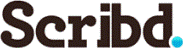

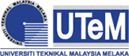
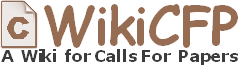
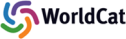
Decoding Twitter: Sentiment Analysis with Machine Learning
Author(s) | Vivek Yadav |
---|---|
Country | India |
Abstract | This research undertakes a comprehensive examination of sentiment analysis on Twitter, leveraging the power of machine learning methodologies. With a focus on decoding the intricate landscape of emotions within the Twitterverse, the study aims to provide valuable insights into understanding sentiments expressed in this dynamic social media platform. The primary objective is to employ machine learning techniques to unravel the underlying sentiments, encompassing positive, negative, and neutral tones within the brevity of Twitter communication. The methodology involves the collection of a diverse dataset of tweets, followed by meticulous preprocessing steps to handle noise, eliminate irrelevant information, and perform tokenization. Feature extraction techniques, such as TF-IDF, are employed to convert textual data into numerical vectors, facilitating the subsequent application of various machine learning models. These models, ranging from traditional approaches like Naive Bayes to advanced ones like Support Vector Machines, are implemented and rigorously evaluated based on key performance metrics such as accuracy, precision, recall, and F1 score. |
Keywords | Sentiment Analysis, Twitter, Machine Learning, , Social Media Analytics |
Field | Computer Applications |
Published In | Volume 6, Issue 1, January-February 2024 |
Published On | 2024-01-20 |
Cite This | Decoding Twitter: Sentiment Analysis with Machine Learning - Vivek Yadav - IJFMR Volume 6, Issue 1, January-February 2024. DOI 10.36948/ijfmr.2024.v06i01.12249 |
DOI | https://doi.org/10.36948/ijfmr.2024.v06i01.12249 |
Short DOI | https://doi.org/gtfmqv |
Share this

E-ISSN 2582-2160

CrossRef DOI is assigned to each research paper published in our journal.
IJFMR DOI prefix is
10.36948/ijfmr
Downloads
All research papers published on this website are licensed under Creative Commons Attribution-ShareAlike 4.0 International License, and all rights belong to their respective authors/researchers.
