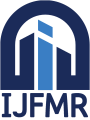
International Journal For Multidisciplinary Research
E-ISSN: 2582-2160
•
Impact Factor: 9.24
A Widely Indexed Open Access Peer Reviewed Multidisciplinary Bi-monthly Scholarly International Journal
Home
Research Paper
Submit Research Paper
Publication Guidelines
Publication Charges
Upload Documents
Track Status / Pay Fees / Download Publication Certi.
Editors & Reviewers
View All
Join as a Reviewer
Get Membership Certificate
Current Issue
Publication Archive
Conference
Publishing Conf. with IJFMR
Upcoming Conference(s) ↓
WSMCDD-2025
GSMCDD-2025
Conferences Published ↓
RBS:RH-COVID-19 (2023)
ICMRS'23
PIPRDA-2023
Contact Us
Plagiarism is checked by the leading plagiarism checker
Call for Paper
Volume 7 Issue 2
March-April 2025
Indexing Partners






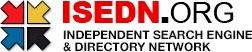

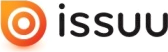


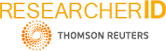
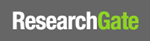
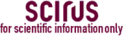
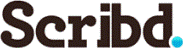

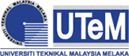
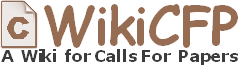
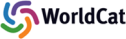
Breakeven Estimation of Solar Energy: A Machine Learning and Time Series Analysis Approach
Author(s) | Amancha Ashwith, Argula Sujith, Kallem Sai Kiran Reddy, Shruthi Kansal |
---|---|
Country | India |
Abstract | This study focuses on determining the breakeven point for solar panel installations, utilizing a comprehensive dataset with daily information like sunrise, sunset, and temperature. Users input parameters such as panel area, location, current cost, and installation charges. Using advanced time series analysis, the system considers environmental factors, energy potential, and local prices to predict when cumulative income will surpass installation costs. This analysis is crucial for individuals and organizations assessing the financial viability of solar investments. By providing insights into profitability timelines, stakeholders can make informed decisions, promoting a sustainable transition to renewable energy. The study aligns with the broader goal of reducing reliance on non-renewable sources and fostering environmentally responsible practices. |
Keywords | Solar radiation, daily energy generation estimation, machine learning, random forest, time series analysis, ARIMA, SARIMA, renewable energy, energy price forecasting. |
Field | Computer > Data / Information |
Published In | Volume 6, Issue 1, January-February 2024 |
Published On | 2024-01-27 |
DOI | https://doi.org/10.36948/ijfmr.2024.v06i01.12551 |
Short DOI | https://doi.org/gtghnb |
Share this

E-ISSN 2582-2160

CrossRef DOI is assigned to each research paper published in our journal.
IJFMR DOI prefix is
10.36948/ijfmr
Downloads
All research papers published on this website are licensed under Creative Commons Attribution-ShareAlike 4.0 International License, and all rights belong to their respective authors/researchers.
