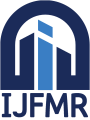
International Journal For Multidisciplinary Research
E-ISSN: 2582-2160
•
Impact Factor: 9.24
A Widely Indexed Open Access Peer Reviewed Multidisciplinary Bi-monthly Scholarly International Journal
Home
Research Paper
Submit Research Paper
Publication Guidelines
Publication Charges
Upload Documents
Track Status / Pay Fees / Download Publication Certi.
Editors & Reviewers
View All
Join as a Reviewer
Get Membership Certificate
Current Issue
Publication Archive
Conference
Publishing Conf. with IJFMR
Upcoming Conference(s) ↓
WSMCDD-2025
GSMCDD-2025
AIMAR-2025
Conferences Published ↓
ICCE (2025)
RBS:RH-COVID-19 (2023)
ICMRS'23
PIPRDA-2023
Contact Us
Plagiarism is checked by the leading plagiarism checker
Call for Paper
Volume 7 Issue 4
July-August 2025
Indexing Partners






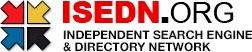

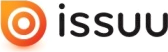


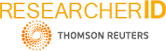
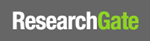
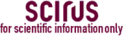
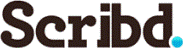

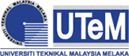
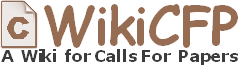
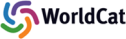
Anomaly Detection in CCTV Surveillance
Author(s) | Shefali Goyal, Arnav Malhotra, Nikita Jain, Karuna Middha |
---|---|
Country | India |
Abstract | CCTV surveillance systems are routinely utilized to maintain the safety and security of public and private locations. However, manually watching surveillance footage may be tiresome and time-consuming, making it difficult to recognize and respond to possible threats quickly. In this research, we offer a real-time danger detection system for CCTV monitoring that uses deep learning models to identify and categorize degrees of high movement in video frames. Our system is able to continuously monitor surveillance footage in real-time and identify potential threats such as abuse, burglaries, explosions, shootings, fighting, shoplifting, road accidents, arson, robbery, stealing, assault, and vandalism by treating videos as segments and defining anomalous (threatening) and normal (safe) segments. We conducted extensive tests on a huge collection of CCTV video to evaluate the effectiveness of our system and obtained encouraging findings. Our solution has the potential to greatly increase the efficiency and efficacy of CCTV surveillance, allowing for faster reaction times and improved individual security. We use multiple instance learning (MIL) to automatically develop a deep anomaly ranking model that predicts high anomaly scores for anomalous video segments by treating normal and anomalous films as bags and video segments as instances. In addition, we apply sparsity and temporal smoothness requirements in the ranking loss function to improve anomaly localization during training. In addition, we present a novel large-scale, first-of-its-kind dataset comprising 128 hours of video. It comprises of 1900 uncut real-world surveillance movies with 13 actual anomalies such as fights, car accidents, burglary, robbery, and so on, as well as typical activities. This dataset may be used for two different purposes. First, global anomaly detection, which takes into account all abnormalities in one group and all normal activity in another. Secondly, for identifying every one of the thirteen unusual actions. Comparing our experimental results to state-of-the-art methodologies, we find that our MIL method for anomaly detection produces a considerable increase on anomaly detection performance. We provide the findings from many recent deep learning baselines concerning the identification of aberrant behavior. These baselines' poor recognition performance indicates how difficult our dataset is, and it also provides additional room for investigation in the future. |
Field | Computer > Artificial Intelligence / Simulation / Virtual Reality |
Published In | Volume 6, Issue 1, January-February 2024 |
Published On | 2024-02-05 |
DOI | https://doi.org/10.36948/ijfmr.2024.v06i01.12750 |
Short DOI | https://doi.org/gtg6rp |
Share this

E-ISSN 2582-2160

CrossRef DOI is assigned to each research paper published in our journal.
IJFMR DOI prefix is
10.36948/ijfmr
Downloads
All research papers published on this website are licensed under Creative Commons Attribution-ShareAlike 4.0 International License, and all rights belong to their respective authors/researchers.
