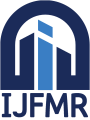
International Journal For Multidisciplinary Research
E-ISSN: 2582-2160
•
Impact Factor: 9.24
A Widely Indexed Open Access Peer Reviewed Multidisciplinary Bi-monthly Scholarly International Journal
Home
Research Paper
Submit Research Paper
Publication Guidelines
Publication Charges
Upload Documents
Track Status / Pay Fees / Download Publication Certi.
Editors & Reviewers
View All
Join as a Reviewer
Get Membership Certificate
Current Issue
Publication Archive
Conference
Publishing Conf. with IJFMR
Upcoming Conference(s) ↓
WSMCDD-2025
GSMCDD-2025
Conferences Published ↓
RBS:RH-COVID-19 (2023)
ICMRS'23
PIPRDA-2023
Contact Us
Plagiarism is checked by the leading plagiarism checker
Call for Paper
Volume 7 Issue 2
March-April 2025
Indexing Partners






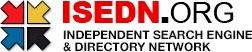

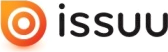


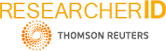
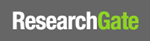
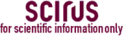
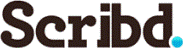

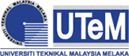
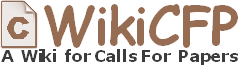
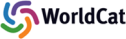
STA-STF based Recurrent Neural Network for Next Location Prediction
Author(s) | Niharika Dinesh, Atul Pandey, Shrey, Shashikiran kummari, Priyanka Jadhav |
---|---|
Country | India |
Abstract | In the evolving landscape of technology and smart devices, the focus on modeling spatial correlations, temporal dynamics, and friendship influence in point-of-interest (POI) check-ins has intensified. Existing works center on capturing user check-in behavior, emphasizing spatial and temporal dependencies of POIs. Markov chain-based methods address instance-level interactions, while recurrent neural network (RNN) approaches excel in handling variable-length check-in sequences. However, the former struggles with high-order POI transition dependency, and the latter cannot discern individual POI contributions in a historical check-in sequence. Additionally, RNNs propagate local and global information through a single bottleneck—hidden states. To address these limitations, a novel model is presented, enforcing contextual constraints on sequential data. The design incorporates spatial and temporal attention mechanisms over an RNN, highlighting the significance of POIs visited by users within specific time intervals and geographical distances during successive check-ins. The attention mechanism aids in identifying crucial POIs based on time difference and spatial distance in user check-in history for predicting the next POI. Periodicity and friendship influence are also considered in the model design. Experimental results on the BrightKite location-based social network demonstrate the proposed method's outperformance over existing state-of-the-art deep neural network methods for the next POI prediction and understanding of user transition behavior. Sensitivity analyses of parameters, including context windows for capturing sequential effects and estimating temporal and spatial attention, further validate the model's effectiveness. In parallel, this paper introduces a Space-Time Features-based Recurrent Neural Network (STF-RNN) for predicting individuals' next movements using mobility patterns from GPS device logs. Unlike traditional approaches, the STF-RNN automatically extracts internal representations of space and time features, enhancing efficiency in uncovering valuable insights into human behavior. Leveraging the sequence-representing ability of RNN structures, the model keeps track of user movement history, enabling the discovery of more meaningful dependencies and, consequently, improving performance. Collectively, these contributions advance the understanding and prediction capabilities in the realms of spatial dynamics, temporal dependencies, and human mobility patterns. |
Keywords | Space-Time Features, Spatio Temporal Modeling, Next Location Prediction, User Transition Behavior |
Field | Engineering |
Published In | Volume 6, Issue 1, January-February 2024 |
Published On | 2024-01-30 |
DOI | https://doi.org/10.36948/ijfmr.2024.v06i01.12754 |
Short DOI | https://doi.org/gtghkh |
Share this

E-ISSN 2582-2160

CrossRef DOI is assigned to each research paper published in our journal.
IJFMR DOI prefix is
10.36948/ijfmr
Downloads
All research papers published on this website are licensed under Creative Commons Attribution-ShareAlike 4.0 International License, and all rights belong to their respective authors/researchers.
