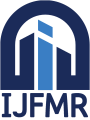
International Journal For Multidisciplinary Research
E-ISSN: 2582-2160
•
Impact Factor: 9.24
A Widely Indexed Open Access Peer Reviewed Multidisciplinary Bi-monthly Scholarly International Journal
Home
Research Paper
Submit Research Paper
Publication Guidelines
Publication Charges
Upload Documents
Track Status / Pay Fees / Download Publication Certi.
Editors & Reviewers
View All
Join as a Reviewer
Reviewer Referral Program
Get Membership Certificate
Current Issue
Publication Archive
Conference
Publishing Conf. with IJFMR
Upcoming Conference(s) ↓
WSMCDD-2025
Conferences Published ↓
RBS:RH-COVID-19 (2023)
ICMRS'23
PIPRDA-2023
Contact Us
Plagiarism is checked by the leading plagiarism checker
Call for Paper
Volume 6 Issue 4
July-August 2024
Indexing Partners






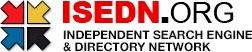

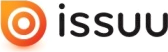


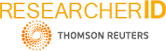
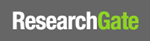
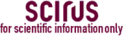
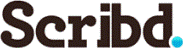

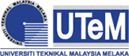
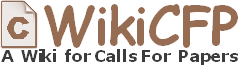
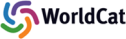
Machine Learning Methods with Link-Level Features
Author(s) | Koffka Khan |
---|---|
Country | Trinidad and Tobago |
Abstract | This paper describes graph link level features. We describe three different kinds of them. We discuss distance-based characteristics that consumers might use, such as the shortest path between two nodes, which does not account for neighborhood overlaps. Then we discuss neighborhood overlap metrics like Common neighbors, Jaccard coefficient, and the Adamic-Adar index that measures how many neighbors a pair of nodes share in a fine-grained manner. The issue with this is that the metric will return a value of 0 for nodes that are more than two hops away or that do not have any neighbors. The global neighborhood overlap metrics, for instance, like Katz use the global graph structure to give us a score for a pair of nodes, and the Katz index counts the number of pets of all lands between a pair of nodes where these paths are discounted exponentially with their length. |
Keywords | graph, link, features, common neighbors, Jaccard coefficient, Adamic-Adar index, Katz index |
Field | Engineering |
Published In | Volume 4, Issue 6, November-December 2022 |
Published On | 2022-12-30 |
Cite This | Machine Learning Methods with Link-Level Features - Koffka Khan - IJFMR Volume 4, Issue 6, November-December 2022. DOI 10.36948/ijfmr.2022.v04i06.1281 |
DOI | https://doi.org/10.36948/ijfmr.2022.v04i06.1281 |
Short DOI | https://doi.org/grkbwf |
Share this
E-ISSN 2582-2160

CrossRef DOI is assigned to each research paper published in our journal.
IJFMR DOI prefix is
10.36948/ijfmr
Downloads
All research papers published on this website are licensed under Creative Commons Attribution-ShareAlike 4.0 International License, and all rights belong to their respective authors/researchers.
