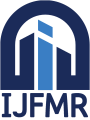
International Journal For Multidisciplinary Research
E-ISSN: 2582-2160
•
Impact Factor: 9.24
A Widely Indexed Open Access Peer Reviewed Multidisciplinary Bi-monthly Scholarly International Journal
Home
Research Paper
Submit Research Paper
Publication Guidelines
Publication Charges
Upload Documents
Track Status / Pay Fees / Download Publication Certi.
Editors & Reviewers
View All
Join as a Reviewer
Reviewer Referral Program
Get Membership Certificate
Current Issue
Publication Archive
Conference
Publishing Conf. with IJFMR
Upcoming Conference(s) ↓
WSMCDD-2025
Conferences Published ↓
RBS:RH-COVID-19 (2023)
ICMRS'23
PIPRDA-2023
Contact Us
Plagiarism is checked by the leading plagiarism checker
Call for Paper
Volume 6 Issue 4
July-August 2024
Indexing Partners






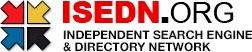

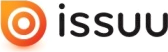


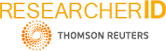
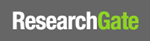
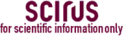
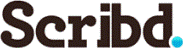

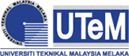
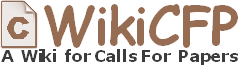
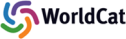
Enhancing Fraud Detection in Financial Statements with Deep Learning: An Audit Perspective
Author(s) | Intissar Grissa, Ezzeddine Abaoub |
---|---|
Country | Tunisia |
Abstract | Financial fraud persists as a formidable challenge, necessitating continuous audit innovations to uphold financial statement integrity. This study explores the application of Deep Learning (DL) techniques in bolstering fraud detection within financial audits. It emphasizes the pivotal role of audits in preserving trust and transparency in business. Highlighting the evolving nature of financial fraud, the study underscores the need for auditors to adapt to sophisticated schemes. An examination of DL methodologies reveals the potential of neural networks, anomaly detection, and predictive modeling in uncovering hidden fraudulent activities. The discourse encompasses data-driven strategies, model architectures, and tailored feature engineering. Real-world case studies demonstrate how DL-driven fraud detection enhances traditional methods by improving accuracy and reducing false positives. The study stresses the importance of continuous monitoring, proactive risk mitigation, and timely fraud prevention. Additionally, it addresses ethical and regulatory considerations, advocating for transparency and responsible AI practices in auditing. In conclusion, this study serves as a valuable resource for auditors and regulators, highlighting the transformative impact of DL in fortifying fraud detection and preserving financial reporting integrity. |
Keywords | Deep Learning, Fraud detection, Financial statements, Audit perspective, Anomaly detection, Regulatory compliance, Responsible AI. |
Field | Business Administration |
Published In | Volume 6, Issue 1, January-February 2024 |
Published On | 2024-02-28 |
Cite This | Enhancing Fraud Detection in Financial Statements with Deep Learning: An Audit Perspective - Intissar Grissa, Ezzeddine Abaoub - IJFMR Volume 6, Issue 1, January-February 2024. DOI 10.36948/ijfmr.2024.v06i01.13451 |
DOI | https://doi.org/10.36948/ijfmr.2024.v06i01.13451 |
Short DOI | https://doi.org/gtktjr |
Share this
E-ISSN 2582-2160

CrossRef DOI is assigned to each research paper published in our journal.
IJFMR DOI prefix is
10.36948/ijfmr
Downloads
All research papers published on this website are licensed under Creative Commons Attribution-ShareAlike 4.0 International License, and all rights belong to their respective authors/researchers.
