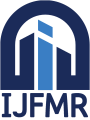
International Journal For Multidisciplinary Research
E-ISSN: 2582-2160
•
Impact Factor: 9.24
A Widely Indexed Open Access Peer Reviewed Multidisciplinary Bi-monthly Scholarly International Journal
Home
Research Paper
Submit Research Paper
Publication Guidelines
Publication Charges
Upload Documents
Track Status / Pay Fees / Download Publication Certi.
Editors & Reviewers
View All
Join as a Reviewer
Reviewer Referral Program
Get Membership Certificate
Current Issue
Publication Archive
Conference
Publishing Conf. with IJFMR
Upcoming Conference(s) ↓
WSMCDD-2025
GSMCDD-2025
Conferences Published ↓
RBS:RH-COVID-19 (2023)
ICMRS'23
PIPRDA-2023
Contact Us
Plagiarism is checked by the leading plagiarism checker
Call for Paper
Volume 6 Issue 6
November-December 2024
Indexing Partners






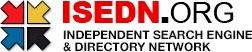

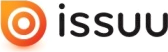


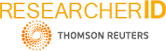
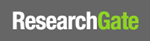
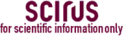
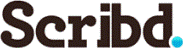

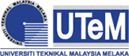
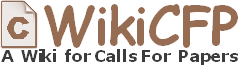
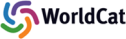
Machine Learning for Anomaly Detection in Accounting Records: A Comprehensive Study
Author(s) | Intissar Grissa, Ezzeddine Abaoub |
---|---|
Country | Tunisia |
Abstract | Maintaining the integrity of accounting records is vital for financial transparency, regulatory compliance, and fraud prevention. This study explores the application of Machine Learning (ML) techniques for anomaly detection in accounting records, shedding light on the evolving landscape of financial data analysis. It emphasizes the critical role of accurate accounting records in business operations, financial reporting, and regulatory adherence. Amidst increasing transaction complexity, traditional audit methods face challenges in detecting unnoticed irregularities. A detailed examination of ML methodologies, including clustering, classification, and neural networks, showcases their potential in identifying anomalies within accounting data. The study discusses data preprocessing, feature engineering, model selection, and evaluation criteria essential for robust anomaly detection. Real-world case studies illustrate how ML-driven anomaly detection enhances traditional accounting practices, improving accuracy and efficiency. It underscores ML's proactive role in preventing fraud, errors, and compliance breaches. Ethical and regulatory considerations in ML implementation are addressed, highlighting the importance of transparency, accountability, and responsible AI practices. This study serves as a valuable resource for accounting professionals and regulatory authorities, emphasizing ML's transformative impact on maintaining financial accuracy and regulatory compliance. |
Keywords | Machine Learning, Anomaly detection, Accounting records, Financial transparency, Fraud prevention, Compliance, Responsible AI. |
Field | Business Administration |
Published In | Volume 6, Issue 1, January-February 2024 |
Published On | 2024-02-27 |
Cite This | Machine Learning for Anomaly Detection in Accounting Records: A Comprehensive Study - Intissar Grissa, Ezzeddine Abaoub - IJFMR Volume 6, Issue 1, January-February 2024. DOI 10.36948/ijfmr.2024.v06i01.13477 |
DOI | https://doi.org/10.36948/ijfmr.2024.v06i01.13477 |
Short DOI | https://doi.org/gtktjq |
Share this

E-ISSN 2582-2160

CrossRef DOI is assigned to each research paper published in our journal.
IJFMR DOI prefix is
10.36948/ijfmr
Downloads
All research papers published on this website are licensed under Creative Commons Attribution-ShareAlike 4.0 International License, and all rights belong to their respective authors/researchers.
