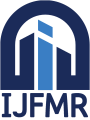
International Journal For Multidisciplinary Research
E-ISSN: 2582-2160
•
Impact Factor: 9.24
A Widely Indexed Open Access Peer Reviewed Multidisciplinary Bi-monthly Scholarly International Journal
Home
Research Paper
Submit Research Paper
Publication Guidelines
Publication Charges
Upload Documents
Track Status / Pay Fees / Download Publication Certi.
Editors & Reviewers
View All
Join as a Reviewer
Get Membership Certificate
Current Issue
Publication Archive
Conference
Publishing Conf. with IJFMR
Upcoming Conference(s) ↓
WSMCDD-2025
GSMCDD-2025
AIMAR-2025
Conferences Published ↓
ICCE (2025)
RBS:RH-COVID-19 (2023)
ICMRS'23
PIPRDA-2023
Contact Us
Plagiarism is checked by the leading plagiarism checker
Call for Paper
Volume 7 Issue 4
July-August 2025
Indexing Partners






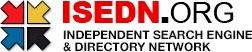

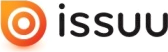


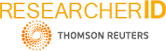
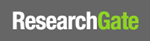
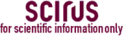
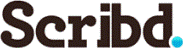

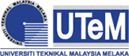
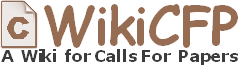
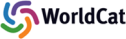
Predicting Cancer Risk from Genome Data: A Multilayer Perceptron Approach
Author(s) | Shreyas Hegde, Vinay Kumar |
---|---|
Country | India |
Abstract | This paper proposes a deep learning method to predict cancer risk from gene symbols using a multilayer perceptron (MLP) feed forward neural network. The paper uses a data set of gene symbols and their corresponding cancer risk labels, obtained from a DNA microarray analysis. The paper then builds and compares different machine learning models, such as logistic regression, linear discriminant analysis, quadratic discriminant analysis, decision tree classifier, gaussian nb, ada boost classifier, gaussian process classifier, support vector machine, and random forest. Deep learning MLP model is built, tuned and optimized for hyperparameters which improves the accuracy significantly by 9.09% compared to the best machine learning model. The paper evaluates the performance of the MLP model on the data set using accuracy, precision, recall, and F1-score metrics. This paper contributes to the field of machine learning and bioinformatics by providing a novel and effective way to predict cancer risk from gene symbols. |
Keywords | Multilayer Perceptron, Feed Forward Neural Network, Gene Symbols, Differential Analysis |
Field | Computer > Artificial Intelligence / Simulation / Virtual Reality |
Published In | Volume 6, Issue 1, January-February 2024 |
Published On | 2024-02-17 |
DOI | https://doi.org/10.36948/ijfmr.2024.v06i01.13568 |
Short DOI | https://doi.org/gtjtzn |
Share this

E-ISSN 2582-2160

CrossRef DOI is assigned to each research paper published in our journal.
IJFMR DOI prefix is
10.36948/ijfmr
Downloads
All research papers published on this website are licensed under Creative Commons Attribution-ShareAlike 4.0 International License, and all rights belong to their respective authors/researchers.
