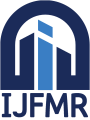
International Journal For Multidisciplinary Research
E-ISSN: 2582-2160
•
Impact Factor: 9.24
A Widely Indexed Open Access Peer Reviewed Multidisciplinary Bi-monthly Scholarly International Journal
Home
Research Paper
Submit Research Paper
Publication Guidelines
Publication Charges
Upload Documents
Track Status / Pay Fees / Download Publication Certi.
Editors & Reviewers
View All
Join as a Reviewer
Get Membership Certificate
Current Issue
Publication Archive
Conference
Publishing Conf. with IJFMR
Upcoming Conference(s) ↓
WSMCDD-2025
GSMCDD-2025
Conferences Published ↓
RBS:RH-COVID-19 (2023)
ICMRS'23
PIPRDA-2023
Contact Us
Plagiarism is checked by the leading plagiarism checker
Call for Paper
Volume 7 Issue 2
March-April 2025
Indexing Partners






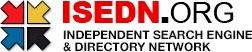

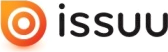


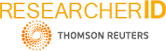
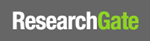
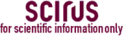
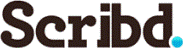

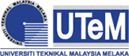
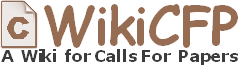
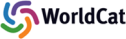
Anomaly Detection in Heterogeneous Data Using Graph Convolutional Networks
Author(s) | Satnam Singh, Shubham Kumar, Ashish Sharma, Shivam, Aarti |
---|---|
Country | India |
Abstract | Closed-circuit television (CCTV) surveillance systems are ubiquitous tools for ensuring public and private safety. However, manual monitoring of surveillance footage is arduous and time-consuming, often resulting in delayed identification and response to potential threats. In this paper, we present a novel real-time threat detection system tailored for CCTV surveillance, employing cutting-edge deep learning methodologies, particularly graph convolutional networks (GCNs), to detect and classify high-movement levels within video frames. By treating videos as segments and delineating between anomalous (potentially threatening) and normal (safe) segments, our system facilitates continuous real-time monitoring of surveillance footage, enabling the timely identification of potential threats such as abuse, burglaries, explosions, shootings, fighting, shoplifting, road accidents, arson, robbery, stealing, assault, and vandalism. To assess the efficacy of our system, we conducted extensive experiments on a large-scale dataset comprising CCTV footage, yielding promising results. Additionally, we introduce a novel, comprehensive dataset comprising 128 hours of real-world surveillance videos, encompassing various anomalies and normal activities. This dataset serves dual purposes: general anomaly detection, and the recognition of specific anomalous activities. Our experimental findings demonstrate the superior performance of our method in anomaly detection compared to state-of-the-art approaches. Furthermore, we provide an analysis of several recent deep learning baselines for anomalous activity recognition, revealing the challenging nature of our dataset and the potential it holds for future research endeavors. Through the integration of advanced deep learning techniques and comprehensive dataset creation, our proposed system stands to significantly enhance the efficiency and effectiveness of CCTV surveillance, facilitating faster response times and bolstering security for individuals in diverse environments. |
Keywords | graph convolutional networks (GCNs), Anamoly Detection |
Field | Computer > Data / Information |
Published In | Volume 6, Issue 1, January-February 2024 |
Published On | 2024-02-27 |
DOI | https://doi.org/10.36948/ijfmr.2024.v06i01.13931 |
Short DOI | https://doi.org/gtkth4 |
Share this

E-ISSN 2582-2160

CrossRef DOI is assigned to each research paper published in our journal.
IJFMR DOI prefix is
10.36948/ijfmr
Downloads
All research papers published on this website are licensed under Creative Commons Attribution-ShareAlike 4.0 International License, and all rights belong to their respective authors/researchers.
