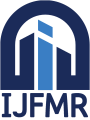
International Journal For Multidisciplinary Research
E-ISSN: 2582-2160
•
Impact Factor: 9.24
A Widely Indexed Open Access Peer Reviewed Multidisciplinary Bi-monthly Scholarly International Journal
Home
Research Paper
Submit Research Paper
Publication Guidelines
Publication Charges
Upload Documents
Track Status / Pay Fees / Download Publication Certi.
Editors & Reviewers
View All
Join as a Reviewer
Get Membership Certificate
Current Issue
Publication Archive
Conference
Publishing Conf. with IJFMR
Upcoming Conference(s) ↓
WSMCDD-2025
GSMCDD-2025
Conferences Published ↓
RBS:RH-COVID-19 (2023)
ICMRS'23
PIPRDA-2023
Contact Us
Plagiarism is checked by the leading plagiarism checker
Call for Paper
Volume 7 Issue 2
March-April 2025
Indexing Partners






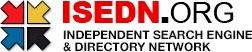

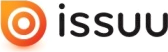


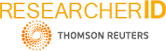
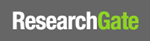
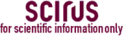
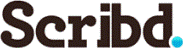

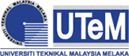
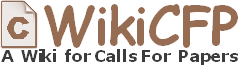
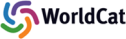
Enhancing Cancer Classification Through Ensemble Machine Learning and Gene Selection Approaches
Author(s) | Omar Gheni Abdulateef |
---|---|
Country | Iraq |
Abstract | The vast dimensionality of gene expression data and the limited number of relevant genes necessitate the adoption of gene selection techniques. Furthermore, the choice of an efficient classifier plays a pivotal role in achieving accurate results. In this study, we employ the Minimum Redundancy Maximum Relevance (mRMR) method for gene selection, coupled with ensemble classifiers and individual classifiers like K-Nearest Neighbors (KNN) and Decision Trees (DT). A comparative analysis between two ensemble classifiers and two individual classifiers is conducted, revealing the superior performance of the ensemble classifiers. Our investigation utilizes four distinct cancer gene expression datasets to showcase the efficacy of employing ensemble classifiers and gene selection methods for cancer classification. The ensemble classifier (Bagging Classifier), in conjunction with the MRMR method selecting only the top 30 genes, achieved an impressive overall accuracy of 94% across all four employed datasets. |
Keywords | Gene Expression, Machine Learning, Gene Selection, Cancer Classification. |
Field | Computer Applications |
Published In | Volume 6, Issue 2, March-April 2024 |
Published On | 2024-03-05 |
DOI | https://doi.org/10.36948/ijfmr.2024.v06i02.14179 |
Short DOI | https://doi.org/gtmbkw |
Share this

E-ISSN 2582-2160

CrossRef DOI is assigned to each research paper published in our journal.
IJFMR DOI prefix is
10.36948/ijfmr
Downloads
All research papers published on this website are licensed under Creative Commons Attribution-ShareAlike 4.0 International License, and all rights belong to their respective authors/researchers.
