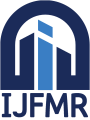
International Journal For Multidisciplinary Research
E-ISSN: 2582-2160
•
Impact Factor: 9.24
A Widely Indexed Open Access Peer Reviewed Multidisciplinary Bi-monthly Scholarly International Journal
Home
Research Paper
Submit Research Paper
Publication Guidelines
Publication Charges
Upload Documents
Track Status / Pay Fees / Download Publication Certi.
Editors & Reviewers
View All
Join as a Reviewer
Get Membership Certificate
Current Issue
Publication Archive
Conference
Publishing Conf. with IJFMR
Upcoming Conference(s) ↓
WSMCDD-2025
GSMCDD-2025
AIMAR-2025
Conferences Published ↓
ICCE (2025)
RBS:RH-COVID-19 (2023)
ICMRS'23
PIPRDA-2023
Contact Us
Plagiarism is checked by the leading plagiarism checker
Call for Paper
Volume 7 Issue 4
July-August 2025
Indexing Partners






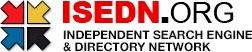

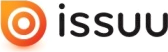


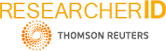
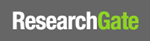
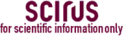
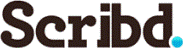

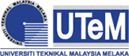
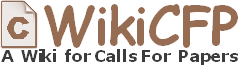
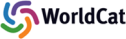
Quantitative Analysis and Forecasting of Industrial CO2 Emissions using Multiple Machine Learning Models
Author(s) | Neev Goenka |
---|---|
Country | India |
Abstract | In response to escalating climate concerns, precise industrial Carbon Dioxide (CO2) emissions prediction is paramount. Employing advanced Machine Learning (ML) techniques, this study focuses on forecasting industrial CO2 emissions using global data from the Our World In Data Dataset (containing information on annual emissions from cement, coal, flaring, gas, and oil industries). Various regression models including Support Vector Regression (SVR), Linear Regression, and XGBoost were explored, with a primary emphasis on time series forecasting models for yearly CO2 emissions. Leveraging time series forecasting, intricate temporal trends in emissions data are discerned, offering enhanced predictive insights. CO2 prediction literature was reviewed, data collected and preprocessed, and various ML algorithms implemented, followed by hyperparameter tuning. The models, rigorously trained and evaluated, yield accurate emission predictions. Results highlight the superior performances of the Transformer model and the Neural Prophet Library developed by Stanford University in collaboration with Facebook Inc., with RMSE scores of 416.58 and 470.30, impressively low MAPE scores of both 0.01, and relatively lower MAE of 349.07 and 380.40 compared to other tested models. DeepTCN also demonstrates competitive predictive capabilities but falls short of Transformer model and Neural Prophet model accuracy. Traditional models including ARIMA, Naive Forecasting, Auto Regression (AR), Exponential Smoothing, and SARIMA lag in performance compared to both Neural Prophet and Transformer. These findings underscore the promising role of ML in advancing sustainable environmental management and pave the way for subsequent research endeavors. |
Keywords | CO2 emissions, Industrial Emissions, Sustainability, Environmental AI, Machine Learning, Time series forecasting. |
Field | Engineering |
Published In | Volume 6, Issue 3, May-June 2024 |
Published On | 2024-06-30 |
DOI | https://doi.org/10.36948/ijfmr.2024.v06i03.14545 |
Short DOI | https://doi.org/gt3nkp |
Share this

E-ISSN 2582-2160

CrossRef DOI is assigned to each research paper published in our journal.
IJFMR DOI prefix is
10.36948/ijfmr
Downloads
All research papers published on this website are licensed under Creative Commons Attribution-ShareAlike 4.0 International License, and all rights belong to their respective authors/researchers.
