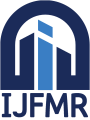
International Journal For Multidisciplinary Research
E-ISSN: 2582-2160
•
Impact Factor: 9.24
A Widely Indexed Open Access Peer Reviewed Multidisciplinary Bi-monthly Scholarly International Journal
Home
Research Paper
Submit Research Paper
Publication Guidelines
Publication Charges
Upload Documents
Track Status / Pay Fees / Download Publication Certi.
Editors & Reviewers
View All
Join as a Reviewer
Get Membership Certificate
Current Issue
Publication Archive
Conference
Publishing Conf. with IJFMR
Upcoming Conference(s) ↓
WSMCDD-2025
GSMCDD-2025
Conferences Published ↓
RBS:RH-COVID-19 (2023)
ICMRS'23
PIPRDA-2023
Contact Us
Plagiarism is checked by the leading plagiarism checker
Call for Paper
Volume 7 Issue 2
March-April 2025
Indexing Partners






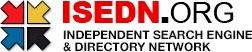

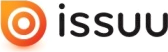


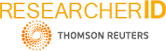
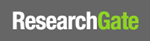
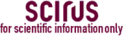
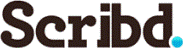

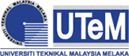
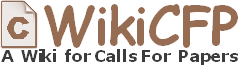
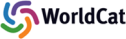
Evolution of Transfer Learning and Generalization in Machine Learning: Advancements Unveiled
Author(s) | C JYOTHI SREE, G RAVI KUMAR, G MAHESH CHALLARI, K VIJAY KUMAR |
---|---|
Country | India |
Abstract | In recent years, transfer learning has emerged as a pivotal technique in machine learning, enabling models to leverage insights gained from one domain to enhance their performance in distinct domains. This shift in approach has catalyzed a surge in research endeavors aimed at uncovering strategies to augment the effectiveness of transfer learning while also addressing the associated challenges. This study delves comprehensively into the multifaceted realm of transfer learning and generalization, exploring a spectrum of methodologies designed to amplify knowledge transfer across diverse contexts. From adapting to varying domains to exploring meta-learning principles, this investigation surveys an array of techniques aimed at bolstering models' capacity to extrapolate their learning to novel tasks and situations. Nevertheless, achieving seamless knowledge transfer encounters challenges such as domain shifts, dataset biases, and high-dimensional data complexities. By critically evaluating these hurdles, this research highlights the impediments that curtail transfer learning's full potential and discusses potential avenues for mitigation. Moreover, in the evolving landscape of machine learning, novel innovations like generative adversarial networks (GANs) and few-shot learning techniques have introduced new dimensions of adaptability and robustness to transfer learning. In summation, this comprehensive exploration underscores the intricate interplay between transfer learning and generalization, contributing to a deeper understanding of effective transfer learning strategies and their associated challenges, while guiding researchers and practitioners to pioneer innovative solutions that advance the frontiers of transfer learning and facilitate the development of more generalized machine learning models. |
Keywords | Transfer Learning, Generalization, Machine Learning, Strategies, Challenges, and Innovations. |
Field | Engineering |
Published In | Volume 6, Issue 2, March-April 2024 |
Published On | 2024-03-23 |
DOI | https://doi.org/10.36948/ijfmr.2024.v06i02.15372 |
Short DOI | https://doi.org/gtn3zd |
Share this

E-ISSN 2582-2160

CrossRef DOI is assigned to each research paper published in our journal.
IJFMR DOI prefix is
10.36948/ijfmr
Downloads
All research papers published on this website are licensed under Creative Commons Attribution-ShareAlike 4.0 International License, and all rights belong to their respective authors/researchers.
