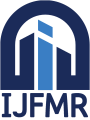
International Journal For Multidisciplinary Research
E-ISSN: 2582-2160
•
Impact Factor: 9.24
A Widely Indexed Open Access Peer Reviewed Multidisciplinary Bi-monthly Scholarly International Journal
Home
Research Paper
Submit Research Paper
Publication Guidelines
Publication Charges
Upload Documents
Track Status / Pay Fees / Download Publication Certi.
Editors & Reviewers
View All
Join as a Reviewer
Get Membership Certificate
Current Issue
Publication Archive
Conference
Publishing Conf. with IJFMR
Upcoming Conference(s) ↓
WSMCDD-2025
GSMCDD-2025
AIMAR-2025
Conferences Published ↓
ICCE (2025)
RBS:RH-COVID-19 (2023)
ICMRS'23
PIPRDA-2023
Contact Us
Plagiarism is checked by the leading plagiarism checker
Call for Paper
Volume 7 Issue 4
July-August 2025
Indexing Partners






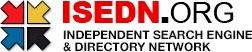

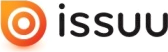


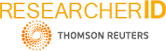
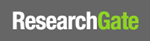
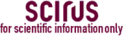
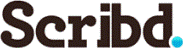

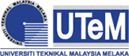
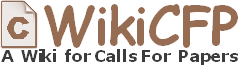
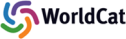
Everything Everywhere All At Once
Author(s) | T. Joshua, S. Madhan, S.V. Girivasan, A. Meenakshi |
---|---|
Country | India |
Abstract | In the era of big data, where vast amounts of information are constantly generated and stored, the ability to efficiently navigate through these datasets and extract meaningful insights has become increasingly crucial. This process, known as data reduction, involves techniques for filtering and condensing large datasets to identify the most relevant and informative data points. By reducing the dimensionality of the data, data reduction facilitates further analysis, interpretation, and visualization, enabling researchers and analysts to gain a deeper understanding of the underlying patterns and trends within the data. One of the primary objectives of data reduction is to improve the effectiveness of basic or fundamental searches. By identifying the data that is most pertinent to these searches, data reduction techniques can significantly enhance the precision and recall of search results. This is particularly valuable in situations where the search criteria are broad or ambiguous, as data reduction can help to narrow down the search space and focus on the most relevant data points. Data reduction techniques can be broadly categorized into two main types: feature selection and dimensionality reduction. Feature selection methods involve identifying and selecting the most relevant subset of features from the original dataset. This process can be performed using various techniques, such as correlation analysis, filter methods, and wrapper methods. Dimensionality reduction techniques, on the other hand, transform the data into a lower-dimensional representation while preserving as much of the original information as possible. |
Keywords | Visualization, Data reduction technique, Dimensionality reduction |
Field | Engineering |
Published In | Volume 6, Issue 2, March-April 2024 |
Published On | 2024-04-04 |
DOI | https://doi.org/10.36948/ijfmr.2024.v06i02.15991 |
Short DOI | https://doi.org/gtp8m9 |
Share this

E-ISSN 2582-2160

CrossRef DOI is assigned to each research paper published in our journal.
IJFMR DOI prefix is
10.36948/ijfmr
Downloads
All research papers published on this website are licensed under Creative Commons Attribution-ShareAlike 4.0 International License, and all rights belong to their respective authors/researchers.
