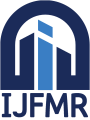
International Journal For Multidisciplinary Research
E-ISSN: 2582-2160
•
Impact Factor: 9.24
A Widely Indexed Open Access Peer Reviewed Multidisciplinary Bi-monthly Scholarly International Journal
Home
Research Paper
Submit Research Paper
Publication Guidelines
Publication Charges
Upload Documents
Track Status / Pay Fees / Download Publication Certi.
Editors & Reviewers
View All
Join as a Reviewer
Reviewer Referral Program
Get Membership Certificate
Current Issue
Publication Archive
Conference
Publishing Conf. with IJFMR
Upcoming Conference(s) ↓
WSMCDD-2025
Conferences Published ↓
RBS:RH-COVID-19 (2023)
ICMRS'23
PIPRDA-2023
Contact Us
Plagiarism is checked by the leading plagiarism checker
Call for Paper
Volume 6 Issue 4
July-August 2024
Indexing Partners






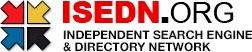

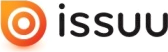


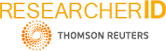
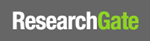
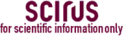
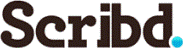

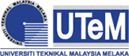
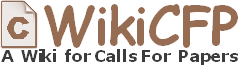
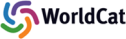
Scalable Cloud Architectures for Distributed Machine Learning: A Comparative Analysis
Author(s) | Lavanya Shanmugam, Kumaran Thirunavukkarasu, Jesu Narkarunai Arasu Malaiyappan, Sanjeev Prakash |
---|---|
Country | United States |
Abstract | This research paper presents a comparative analysis of scalable cloud architectures for distributed machine learning (ML) applications. Through experimentation and evaluation, we investigate key performance metrics including throughput, latency, and resource utilization across three major cloud platforms: Amazon Web Services (AWS), Microsoft Azure, and Google Cloud Platform (GCP). Our findings reveal significant differences in performance among the platforms, with GCP demonstrating superior throughput and lower latency compared to AWS and Azure. Additionally, we analyse resource utilization metrics such as CPU, memory, and storage usage to provide insights into the efficiency of each cloud architecture in supporting ML workloads. By considering both quantitative metrics and qualitative factors, such as ease of deployment and cost-effectiveness, organizations can make informed decisions when selecting a cloud platform for distributed ML applications. |
Keywords | Scalable cloud architectures, distributed machine learning, comparative analysis, throughput, latency, resource utilization, Amazon Web Services, Microsoft Azure, Google Cloud Platform |
Field | Engineering |
Published In | Volume 6, Issue 1, January-February 2024 |
Published On | 2024-01-05 |
Cite This | Scalable Cloud Architectures for Distributed Machine Learning: A Comparative Analysis - Lavanya Shanmugam, Kumaran Thirunavukkarasu, Jesu Narkarunai Arasu Malaiyappan, Sanjeev Prakash - IJFMR Volume 6, Issue 1, January-February 2024. DOI 10.36948/ijfmr.2024.v06i01.16040 |
DOI | https://doi.org/10.36948/ijfmr.2024.v06i01.16040 |
Short DOI | https://doi.org/ |
Share this
E-ISSN 2582-2160

CrossRef DOI is assigned to each research paper published in our journal.
IJFMR DOI prefix is
10.36948/ijfmr
Downloads
All research papers published on this website are licensed under Creative Commons Attribution-ShareAlike 4.0 International License, and all rights belong to their respective authors/researchers.
