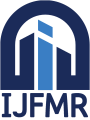
International Journal For Multidisciplinary Research
E-ISSN: 2582-2160
•
Impact Factor: 9.24
A Widely Indexed Open Access Peer Reviewed Multidisciplinary Bi-monthly Scholarly International Journal
Home
Research Paper
Submit Research Paper
Publication Guidelines
Publication Charges
Upload Documents
Track Status / Pay Fees / Download Publication Certi.
Editors & Reviewers
View All
Join as a Reviewer
Get Membership Certificate
Current Issue
Publication Archive
Conference
Publishing Conf. with IJFMR
Upcoming Conference(s) ↓
WSMCDD-2025
GSMCDD-2025
Conferences Published ↓
RBS:RH-COVID-19 (2023)
ICMRS'23
PIPRDA-2023
Contact Us
Plagiarism is checked by the leading plagiarism checker
Call for Paper
Volume 7 Issue 2
March-April 2025
Indexing Partners






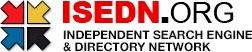

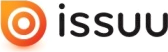


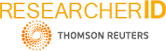
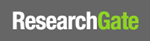
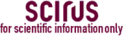
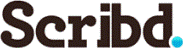

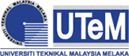
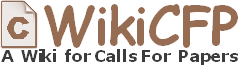
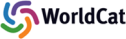
Survival Analysis Based QoS Recommendation for Bus Transportation using Deep Learning
Author(s) | Himabindu N, Dr. Samyama Gunjal G H, Nagendrababu N C |
---|---|
Country | India |
Abstract | Public transportation play an important role in metropolitan cities.Bus services are an important part of public transportation systems, which are frequently chosen because they are convenient, economical, environmentally beneficial, and less taxing on the infrastructure. The temporal patterns of bus transportation incidents are examined in this study using survival analysis, with a focus on the amount of time until certain events like delays, malfunctions, or safe arrivals at destinations. High-dimensional data is being generated at an increasing rate due to technological advancements. As a result, it is quite difficult to analyze such a dataset adequately. Algorithms for machine learning (ML) have become popular tools for modeling complex and nonlinear interactions in a variety of real-world applications, including as the analysis of high-dimensional survival data. Multilayer Deep Neural Network (DNN) models have achieved impressive results recently. Thus, using Keras and TensorFlow, a Cox-based DNN prediction survival model (DNNSurv model) was created. Its findings, however, were limited to survival datasets with large sample sizes or high dimensionality. Using high dimensional survival data, the proposed work will evaluate the DNNSurv model's prediction ability and compare it to a well-known machine learning survival prediction model (random survival forest). The Proposed Work also offers the best settings for a number of hyperparameters, including tuning parameter selection, for this reason. The suggested approach showed through data analysis that, when compared to the ML model, the DNNSurv model performed better overall in terms of the two primary survival prediction evaluation measures (i.e., the concordance index and the time-dependent AUC). |
Keywords | DNNSurv, Deep Neural Networks,Random Survival Forest, Survival Analysis |
Field | Computer |
Published In | Volume 6, Issue 2, March-April 2024 |
Published On | 2024-04-01 |
DOI | https://doi.org/10.36948/ijfmr.2024.v06i02.16062 |
Short DOI | https://doi.org/gtpw7m |
Share this

E-ISSN 2582-2160

CrossRef DOI is assigned to each research paper published in our journal.
IJFMR DOI prefix is
10.36948/ijfmr
Downloads
All research papers published on this website are licensed under Creative Commons Attribution-ShareAlike 4.0 International License, and all rights belong to their respective authors/researchers.
