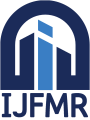
International Journal For Multidisciplinary Research
E-ISSN: 2582-2160
•
Impact Factor: 9.24
A Widely Indexed Open Access Peer Reviewed Multidisciplinary Bi-monthly Scholarly International Journal
Home
Research Paper
Submit Research Paper
Publication Guidelines
Publication Charges
Upload Documents
Track Status / Pay Fees / Download Publication Certi.
Editors & Reviewers
View All
Join as a Reviewer
Get Membership Certificate
Current Issue
Publication Archive
Conference
Publishing Conf. with IJFMR
Upcoming Conference(s) ↓
WSMCDD-2025
GSMCDD-2025
AIMAR-2025
Conferences Published ↓
ICCE (2025)
RBS:RH-COVID-19 (2023)
ICMRS'23
PIPRDA-2023
Contact Us
Plagiarism is checked by the leading plagiarism checker
Call for Paper
Volume 7 Issue 4
July-August 2025
Indexing Partners






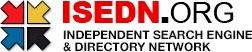

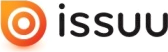


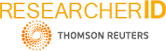
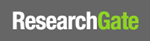
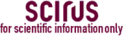
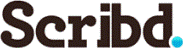

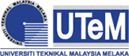
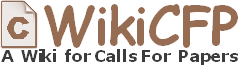
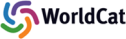
Design and Evaluation of a High-Efficiency Smart IoT Device Incorporating Embedded Tiny Machine Learning for Monitoring Asset Activity
Author(s) | M.K.Kishore, K.Saritha, M.V.N.Chandrika, B.Bhanu sai, Y.Pavan raghava |
---|---|
Country | India |
Abstract | This article suggests a proof-of-concept gadget with an energy-efficient architectural design that can track the use of handheld power tools and identify construction-related activities (like various drilling jobs) as well as possible misuses (like drops). The device's design relies on RFID and WIFI module (ESP8266) communication. Wifi module is used for data exchange with a gateway, whereas RFID is used to identifyy the user. An accelerometer is included for tracking tool use, while sensors for temperature and humidity are used to keep an eye on storage conditions. Information processing at the edge is accomplished by using the Arudino uno that is included within the wifi module. In order to analyze the data directly on board and achieve low latency and great energy efficiency, a Tiny Machine Learning (TinyML) method is developed. The suggested gadget contains an incorporated TinyML algorithm that has been created to identify three distinct use classes: wood drilling, metal drilling, and no-load. The device was coupled to a construction rotary hammer drill to capture a dataset that included over 280 minutes of three-axis accelerations during various operations. This dataset was utilized to train and verify the algorithm. Using a model size of around 30 kB, a neural architecture search was conducted to maximize the trade- off between accuracy and complexity, and an accuracy of 90.6% was obtained. Based on the testing findings, TinyML ran on the edge with an ultralow power usage of 550 nA in sleep mode and an 8 mA peak power consumption. With a basic 250-mAh coin battery, this leads to a balanced mix of low power consumption and edge processing capabilities, allowing the creation of a smart Internet of Things (IoT) device in the field with a long lifespan of up to four years in operation and 17 years in shelf mode. This study closes the gap between edge processing and fine-granularity data assessment by enabling extended battery lifetime operation of device degradation and utility analysis |
Keywords | Asset management, condition monitoring, construction 4.0, energy efficiency, low-power design, smart sensors, tool usage monitoring, wireless sensor networks |
Field | Engineering |
Published In | Volume 6, Issue 2, March-April 2024 |
Published On | 2024-04-18 |
DOI | https://doi.org/10.36948/ijfmr.2024.v06i02.17525 |
Short DOI | https://doi.org/gtrfmg |
Share this

E-ISSN 2582-2160

CrossRef DOI is assigned to each research paper published in our journal.
IJFMR DOI prefix is
10.36948/ijfmr
Downloads
All research papers published on this website are licensed under Creative Commons Attribution-ShareAlike 4.0 International License, and all rights belong to their respective authors/researchers.
