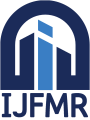
International Journal For Multidisciplinary Research
E-ISSN: 2582-2160
•
Impact Factor: 9.24
A Widely Indexed Open Access Peer Reviewed Multidisciplinary Bi-monthly Scholarly International Journal
Home
Research Paper
Submit Research Paper
Publication Guidelines
Publication Charges
Upload Documents
Track Status / Pay Fees / Download Publication Certi.
Editors & Reviewers
View All
Join as a Reviewer
Reviewer Referral Program
Get Membership Certificate
Current Issue
Publication Archive
Conference
Publishing Conf. with IJFMR
Upcoming Conference(s) ↓
WSMCDD-2025
Conferences Published ↓
RBS:RH-COVID-19 (2023)
ICMRS'23
PIPRDA-2023
Contact Us
Plagiarism is checked by the leading plagiarism checker
Call for Paper
Volume 6 Issue 4
July-August 2024
Indexing Partners






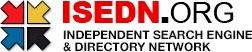

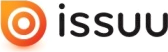


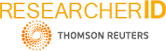
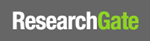
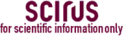
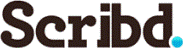

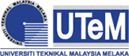
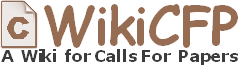
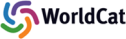
Advanced Neural Network Architecture for Enhanced Multi-Lead ECG Arrhythmia Detection through Optimized Feature Extraction
Author(s) | Bhavith Chandra Challagundla |
---|---|
Country | India |
Abstract | Cardiovascular diseases are a pervasive global health concern, contributing significantly to morbidity and mortality rates worldwide. Among these conditions, arrhythmia, characterized by irregular heart rhythms, presents formidable diagnostic challenges. This study introduces an innovative approach utilizing deep learning techniques, specifically Convolutional Neural Networks (CNNs), to address the complexities of arrhythmia classification. Leveraging multi-lead Electrocardiogram (ECG) data, our CNN model, comprising six layers with a residual block, demonstrates promising outcomes in identifying five distinct heartbeat types: Left Bundle Branch Block (LBBB), Right Bundle Branch Block (RBBB), Atrial Premature Contraction (APC), Premature Ventricular Contraction (PVC), and Normal Beat. Through rigorous experimentation, we highlight the transformative potential of our methodology in enhancing diagnostic accuracy for cardiovascular arrhythmias. Arrhythmia diagnosis remains a critical challenge in cardiovascular care, often relying on manual interpretation of ECG signals, which can be time-consuming and prone to subjectivity. To address these limitations, we propose a novel approach that leverages deep learning algorithms to automate arrhythmia classification. By employing advanced CNN architectures and multi-lead ECG data, our methodology offers a robust solution for precise and efficient arrhythmia detection. Through comprehensive evaluation, we demonstrate the effectiveness of our approach in facilitating more accurate clinical decision-making, thereby improving patient outcomes in managing cardiovascular arrhythmias. |
Keywords | Cardiovascular diseases, Arrhythmia Classification, Deep learning, Convolutional Neural Networks (CNNs), Electrocardiogram (ECG) Analysis, Natural Language Processing (NLP) |
Field | Computer > Artificial Intelligence / Simulation / Virtual Reality |
Published In | Volume 6, Issue 2, March-April 2024 |
Published On | 2024-04-24 |
Cite This | Advanced Neural Network Architecture for Enhanced Multi-Lead ECG Arrhythmia Detection through Optimized Feature Extraction - Bhavith Chandra Challagundla - IJFMR Volume 6, Issue 2, March-April 2024. DOI 10.36948/ijfmr.2024.v06i02.18001 |
DOI | https://doi.org/10.36948/ijfmr.2024.v06i02.18001 |
Short DOI | https://doi.org/gtsg7x |
Share this
E-ISSN 2582-2160

CrossRef DOI is assigned to each research paper published in our journal.
IJFMR DOI prefix is
10.36948/ijfmr
Downloads
All research papers published on this website are licensed under Creative Commons Attribution-ShareAlike 4.0 International License, and all rights belong to their respective authors/researchers.
