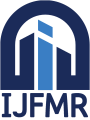
International Journal For Multidisciplinary Research
E-ISSN: 2582-2160
•
Impact Factor: 9.24
A Widely Indexed Open Access Peer Reviewed Multidisciplinary Bi-monthly Scholarly International Journal
Home
Research Paper
Submit Research Paper
Publication Guidelines
Publication Charges
Upload Documents
Track Status / Pay Fees / Download Publication Certi.
Editors & Reviewers
View All
Join as a Reviewer
Get Membership Certificate
Current Issue
Publication Archive
Conference
Publishing Conf. with IJFMR
Upcoming Conference(s) ↓
WSMCDD-2025
GSMCDD-2025
Conferences Published ↓
RBS:RH-COVID-19 (2023)
ICMRS'23
PIPRDA-2023
Contact Us
Plagiarism is checked by the leading plagiarism checker
Call for Paper
Volume 7 Issue 2
March-April 2025
Indexing Partners






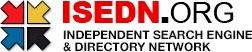

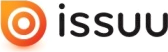


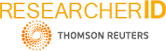
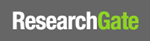
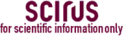
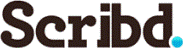

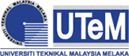
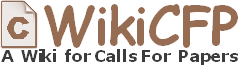
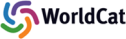
Vision Saver Deep Learning Approaches for Diabetic Retinopathy Detection
Author(s) | Prudhvee Narasimha Goli, Pendela Harshadeep, Asapu Surendra, Muddineni krishna Vamsi |
---|---|
Country | India |
Abstract | One of the main causes of adult blindness and a frequent consequence of diabetes is diabetic retinopathy. In order to preserve eyesight, diabetic retinopathy must be identified and treated early. In this study, "Vision Saver," a deep learning-based method for the automated detection and treatment of diabetic retinopathy, is introduced. Vision Saver provides a comprehensive solution for the detection of retinal problems by utilizing deep neural networks, enabling prompt patient care and intervention. Convolutional neural networks (CNNs) and recurrent neural networks (RNNs), two cutting-edge deep learning approaches, are used by the proposed system to evaluate retinal images and provide precise diagnoses. Additionally, we investigate how generative adversarial networks (GANs) might improve image quality and produce artificial data for training. The architecture of Vision Saver is built to handle both fundus photos and optical coherence tomography (OCT) images, enabling a flexible method of evaluating diabetic retinopathy. Vision Saver's management component includes not only detection but also monitoring of therapy progression. The system aids medical personnel in making educated judgments about interventions like laser therapy, injections, or surgical operations through ongoing monitoring and follow-up. The development and assessment of Vision Saver are covered in this essay, with a focus on how well it performs in terms of sensitivity, specificity, and accuracy. We also discuss concerns with model interpretability, data protection, and integration into clinical workflows. Ultimately, the objective is to improve patient outcomes and lessen the burden of blindness brought on by diabetic retinopathy by offering a comprehensive solution that improves screening and management of the illness. Deep learning in healthcare has a lot of potential, and Vision Saver is an example of how it can significantly improve the lives of people with diabetic retinopathy. |
Keywords | Diabetic Retinopathy, Deep Learning, Detection, Management, Vision Saver, Healthcare Technology |
Field | Engineering |
Published In | Volume 6, Issue 2, March-April 2024 |
Published On | 2024-04-23 |
DOI | https://doi.org/10.36948/ijfmr.2024.v06i02.18176 |
Short DOI | https://doi.org/gtrssg |
Share this

E-ISSN 2582-2160

CrossRef DOI is assigned to each research paper published in our journal.
IJFMR DOI prefix is
10.36948/ijfmr
Downloads
All research papers published on this website are licensed under Creative Commons Attribution-ShareAlike 4.0 International License, and all rights belong to their respective authors/researchers.
