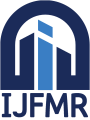
International Journal For Multidisciplinary Research
E-ISSN: 2582-2160
•
Impact Factor: 9.24
A Widely Indexed Open Access Peer Reviewed Multidisciplinary Bi-monthly Scholarly International Journal
Home
Research Paper
Submit Research Paper
Publication Guidelines
Publication Charges
Upload Documents
Track Status / Pay Fees / Download Publication Certi.
Editors & Reviewers
View All
Join as a Reviewer
Get Membership Certificate
Current Issue
Publication Archive
Conference
Publishing Conf. with IJFMR
Upcoming Conference(s) ↓
WSMCDD-2025
GSMCDD-2025
Conferences Published ↓
RBS:RH-COVID-19 (2023)
ICMRS'23
PIPRDA-2023
Contact Us
Plagiarism is checked by the leading plagiarism checker
Call for Paper
Volume 7 Issue 2
March-April 2025
Indexing Partners






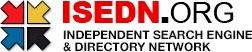

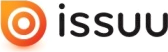


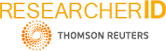
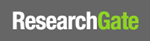
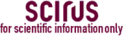
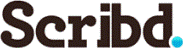

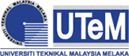
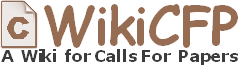
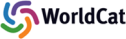
Classification of Autism Spectrum Disorder using Resting State-Functional Magnetic Resonance Imaging and Artificial Neural Network
Author(s) | K. Lakshmipriya, R. Harini, A. Jegadheep Edwin, O. Uma Maheswari |
---|---|
Country | India |
Abstract | Autism Spectrum Disorder (ASD) represents a growing challenge in public health, characterized by irreversible neurodevelopmental abnormalities. Despite a rising prevalence, the underlying aetiology and neural substrates of ASD remain incompletely understood. Resting state-functional Magnetic Resonance Imaging (rs-fMRI) has emerged as a valuable non-invasive tool for probing the organization and cognitive functions of the brain by capturing hemodynamic changes. This study explores the relationship between Functional Connectivity (FC) alterations, measured by rs-fMRI, and the manifestation of ASD-related brain disorders. In this paper, we propose a hybrid approach employing an InfoMax Independent Component Analysis (ICA) algorithm and Artificial Neural Network to distinguish between the ASD subjects and Healthy Controls (HCs). Initially, rs-fMRI datasets are preprocessed using Statistical Parametric Mapping (SPM). Further, these datasets are processed using InfoMax ICA to extract the distinct features such as fractional Amplitude of Low-Frequency Fluctuations (fALFF) and Dynamic Range and visualize the FC alterations by region mapping. In addition to this, the number of activated voxels for each brain regions are estimated to observe the neural abnormalities. Finally, the extracted features are fed as input to train, test and validate the ANN classifier. The efficacy of the proposed network is evaluated using the Autism Brain Imaging Data Exchange (ABIDE) dataset and an accuracy of 75.3 percentage is achieved. |
Keywords | Resting state-functional Magnetic Resonance Imaging, Autism Spectrum Disorder, Functional Connectivity, InfoMax Independent Component Analysis, Artificial Neural Network, Fractional Amplitude of Low-Frequency Fluctuations, Dynamic Range |
Field | Engineering |
Published In | Volume 6, Issue 2, March-April 2024 |
Published On | 2024-04-26 |
DOI | https://doi.org/10.36948/ijfmr.2024.v06i02.18204 |
Short DOI | https://doi.org/gtsg66 |
Share this

E-ISSN 2582-2160

CrossRef DOI is assigned to each research paper published in our journal.
IJFMR DOI prefix is
10.36948/ijfmr
Downloads
All research papers published on this website are licensed under Creative Commons Attribution-ShareAlike 4.0 International License, and all rights belong to their respective authors/researchers.
