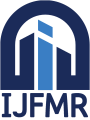
International Journal For Multidisciplinary Research
E-ISSN: 2582-2160
•
Impact Factor: 9.24
A Widely Indexed Open Access Peer Reviewed Multidisciplinary Bi-monthly Scholarly International Journal
Home
Research Paper
Submit Research Paper
Publication Guidelines
Publication Charges
Upload Documents
Track Status / Pay Fees / Download Publication Certi.
Editors & Reviewers
View All
Join as a Reviewer
Reviewer Referral Program
Get Membership Certificate
Current Issue
Publication Archive
Conference
Publishing Conf. with IJFMR
Upcoming Conference(s) ↓
WSMCDD-2025
Conferences Published ↓
RBS:RH-COVID-19 (2023)
ICMRS'23
PIPRDA-2023
Contact Us
Plagiarism is checked by the leading plagiarism checker
Call for Paper
Volume 6 Issue 4
July-August 2024
Indexing Partners






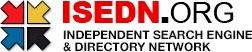

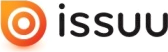


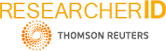
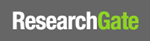
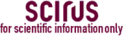
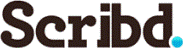

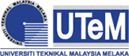
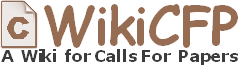
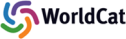
SaleSurf - Strengthening the Web's Armour, One URL at a Time - Malicious URL Detection using Machine Learning Models
Author(s) | Sunidhi Rathod, Nehal Panchal, Atul Sarowa, Prof. Vricha Chavhan |
---|---|
Country | India |
Abstract | One of the most common cybersecurity vulnerabilities involves malicious websites or URLs. Every year, individuals and organizations suffer major financial losses from using harmful content such as spam, malware, inappropriate advertising, and scams that encourage visitors to cheat. These malicious URLs are often promoted through emails, advertisements, web search results, or links to other websites. Considering how many users click on these malicious URLs, there is an urgent need for a reliable system that can classify and identify dangerous URLs; In particular, phishing, spam and malware attacks are increasing. Data volume, updated attack models and strategies, correlation between URL features, lack of data, inconsistent data and the presence of outliers make the division of labor very difficult. In our research, we focus on negative URL search to gain more insight. Our information is divided into four main categories: phishing, harmless (safe), tampering, and malware. We have collected a large database of 651,191 URLs to support our application. To achieve the goal of identifying and identifying malicious URLs, we use three machine learning algorithms: Random Forest, LightGBM, XGBoost, Logistic Regression, CNN and Ensemble Model. |
Keywords | URL discovery, network security, machine learning, URL isolation, phishing, benign, tampering, malware. |
Field | Engineering |
Published In | Volume 6, Issue 2, March-April 2024 |
Published On | 2024-04-25 |
Cite This | SaleSurf - Strengthening the Web's Armour, One URL at a Time - Malicious URL Detection using Machine Learning Models - Sunidhi Rathod, Nehal Panchal, Atul Sarowa, Prof. Vricha Chavhan - IJFMR Volume 6, Issue 2, March-April 2024. DOI 10.36948/ijfmr.2024.v06i02.18240 |
DOI | https://doi.org/10.36948/ijfmr.2024.v06i02.18240 |
Short DOI | https://doi.org/gtsg6w |
Share this
E-ISSN 2582-2160

CrossRef DOI is assigned to each research paper published in our journal.
IJFMR DOI prefix is
10.36948/ijfmr
Downloads
All research papers published on this website are licensed under Creative Commons Attribution-ShareAlike 4.0 International License, and all rights belong to their respective authors/researchers.
