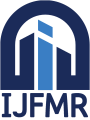
International Journal For Multidisciplinary Research
E-ISSN: 2582-2160
•
Impact Factor: 9.24
A Widely Indexed Open Access Peer Reviewed Multidisciplinary Bi-monthly Scholarly International Journal
Home
Research Paper
Submit Research Paper
Publication Guidelines
Publication Charges
Upload Documents
Track Status / Pay Fees / Download Publication Certi.
Editors & Reviewers
View All
Join as a Reviewer
Reviewer Referral Program
Get Membership Certificate
Current Issue
Publication Archive
Conference
Publishing Conf. with IJFMR
Upcoming Conference(s) ↓
WSMCDD-2025
Conferences Published ↓
RBS:RH-COVID-19 (2023)
ICMRS'23
PIPRDA-2023
Contact Us
Plagiarism is checked by the leading plagiarism checker
Call for Paper
Volume 6 Issue 4
July-August 2024
Indexing Partners






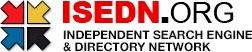

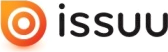


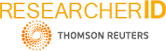
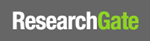
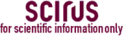
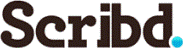

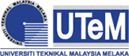
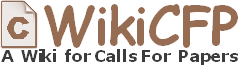
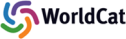
Stock Market Prediction and Analysis using Supervised Learning
Author(s) | Ashutosh Talekar, Anirudha Landage, Lokesh Dhake, Shambhooraje Jadhav, Reshma Kohad |
---|---|
Country | India |
Abstract | The Stock Market Remains a Captivating Subject for Stockbrokers and Investors Seeking Financial Success Through Strategic Equity Trading. Informed Decisions Are Pivotal in Navigating the Dynamic Landscape of Stock Investments, Prompting the Adoption of Various Predictive Techniques. This Study Introduces a Novel Prediction Algorithm That Elucidates the Intricate Relationship Between Independent Variables—Comprising Opening and Closing Prices, High and Low Stock Values, and Trading Volume—and the Dependent Variable, Which Is the Stock Price. Leveraging a Deep Learning System, We Demonstrate the Efficacy of Generating Precise Stock Price Forecasts. Our Research Ambit Encompasses a Comprehensive Exploration of Diverse Deep-Learning Architectures Tailored to Anticipate Stock Prices for Global Conglomerates and Indian Enterprises. A Primary Objective Is to Conduct a Comparative Analysis of These Architectures, Discerning Their Respective Performances in Stock Price Prediction Scenarios. Notably, Long Short-Term Memory (Lstm) Algorithms Are Instrumental in Achieving Heightened Accuracy and Robust Prediction Outcomes. The Methodology Entails Meticulous Data Collection From Historical Stock Market Datasets Spanning Various Companies of Global and Indian Origin. Subsequent Data Preprocessing Involves Addressing Missing Values, Standardizing Features, and Structuring the Data Into Sequences Conducive to Lstm Model Input. The Lstm Architecture, Characterized by Its Adeptness in Capturing Long-Term Dependencies and Temporal Patterns, Forms the Cornerstone of Our Prediction Model. Through This Research Endeavor, We Aim to Provide Valuable Insights Into the Potential of Deep Learning Algorithms, Particularly Lstm, in Facilitating Informed Decision-Making for Investors and Stock Market Participants |
Keywords | Stock Market, Price Prediction, Supervised Learning, Machine Learning, Deep Learning, LSTM. |
Field | Computer |
Published In | Volume 6, Issue 2, March-April 2024 |
Published On | 2024-04-26 |
Cite This | Stock Market Prediction and Analysis using Supervised Learning - Ashutosh Talekar, Anirudha Landage, Lokesh Dhake, Shambhooraje Jadhav, Reshma Kohad - IJFMR Volume 6, Issue 2, March-April 2024. DOI 10.36948/ijfmr.2024.v06i02.18491 |
DOI | https://doi.org/10.36948/ijfmr.2024.v06i02.18491 |
Short DOI | https://doi.org/gtsg49 |
Share this
E-ISSN 2582-2160

CrossRef DOI is assigned to each research paper published in our journal.
IJFMR DOI prefix is
10.36948/ijfmr
Downloads
All research papers published on this website are licensed under Creative Commons Attribution-ShareAlike 4.0 International License, and all rights belong to their respective authors/researchers.
