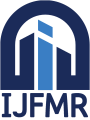
International Journal For Multidisciplinary Research
E-ISSN: 2582-2160
•
Impact Factor: 9.24
A Widely Indexed Open Access Peer Reviewed Multidisciplinary Bi-monthly Scholarly International Journal
Home
Research Paper
Submit Research Paper
Publication Guidelines
Publication Charges
Upload Documents
Track Status / Pay Fees / Download Publication Certi.
Editors & Reviewers
View All
Join as a Reviewer
Get Membership Certificate
Current Issue
Publication Archive
Conference
Publishing Conf. with IJFMR
Upcoming Conference(s) ↓
WSMCDD-2025
GSMCDD-2025
Conferences Published ↓
RBS:RH-COVID-19 (2023)
ICMRS'23
PIPRDA-2023
Contact Us
Plagiarism is checked by the leading plagiarism checker
Call for Paper
Volume 7 Issue 2
March-April 2025
Indexing Partners






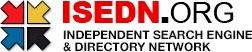

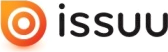


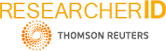
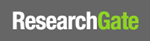
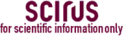
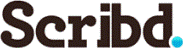

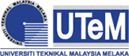
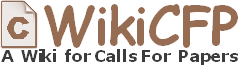
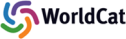
Machine Learning-driven Lung Cancer Detection
Author(s) | PRIYANSHU SAHAY, DEEPANSHU GUPTA, PIYUSH KUMAR, MEDHAVI PANDEY |
---|---|
Country | India |
Abstract | Lung cancer remains one of the most prevalent and deadly forms of cancer worldwide, highlighting the critical need for early detection methods to improve patient outcomes. In this study, we present a novel approach to lung cancer detection utilizing machine learning techniques, specifically leveraging the Random Forest algorithm. Our research focuses on developing a robust and accurate model capable of detecting lung cancer from medical imaging data with high precision and sensitivity. We collected a comprehensive dataset consisting of radiographic images from patients with confirmed lung cancer diagnoses, as well as from healthy individuals for comparison. The Random Forest algorithm, known for its versatility and ability to handle complex datasets, was employed as the primary machine learning framework in our study. Through a process of feature extraction and selection, our model was trained to identify patterns and subtle abnormalities indicative of lung cancer within the imaging data. We conducted extensive experimentation and evaluation to assess the performance of our proposed approach. Our results demonstrate promising outcomes, with the Random Forest model achieving notable accuracy rates in distinguishing between cancerous and non-cancerous lung tissues. Additionally, the model exhibited favorable sensitivity and specificity metrics, indicating its potential as a reliable tool for early lung cancer detection. Furthermore, we conducted comparative analyses with other machine learning algorithms commonly utilized in medical image analysis tasks. The findings underscore the efficacy of the Random Forest algorithm in this particular domain, showcasing its superiority in terms of both performance and interpretability. In conclusion, our research contributes to the ongoing efforts aimed at improving lung cancer diagnosis through the integration of machine learning technologies. By harnessing the power of the Random Forest algorithm, we offer a promising solution for early detection, thereby facilitating timely interventions and ultimately enhancing patient outcomes in the fight against lung cancer. |
Keywords | Lung cancer detection, machine learning, Random Forest algorithm, medical imaging data, feature extraction, model evaluation, sensitivity, specificity, comparative analysis, interpretability, early detection, patient outcomes. |
Field | Engineering |
Published In | Volume 6, Issue 2, March-April 2024 |
Published On | 2024-04-29 |
DOI | https://doi.org/10.36948/ijfmr.2024.v06i02.18531 |
Short DOI | https://doi.org/gtsnv3 |
Share this

E-ISSN 2582-2160

CrossRef DOI is assigned to each research paper published in our journal.
IJFMR DOI prefix is
10.36948/ijfmr
Downloads
All research papers published on this website are licensed under Creative Commons Attribution-ShareAlike 4.0 International License, and all rights belong to their respective authors/researchers.
