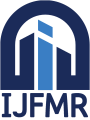
International Journal For Multidisciplinary Research
E-ISSN: 2582-2160
•
Impact Factor: 9.24
A Widely Indexed Open Access Peer Reviewed Multidisciplinary Bi-monthly Scholarly International Journal
Home
Research Paper
Submit Research Paper
Publication Guidelines
Publication Charges
Upload Documents
Track Status / Pay Fees / Download Publication Certi.
Editors & Reviewers
View All
Join as a Reviewer
Reviewer Referral Program
Get Membership Certificate
Current Issue
Publication Archive
Conference
Publishing Conf. with IJFMR
Upcoming Conference(s) ↓
WSMCDD-2025
Conferences Published ↓
RBS:RH-COVID-19 (2023)
ICMRS'23
PIPRDA-2023
Contact Us
Plagiarism is checked by the leading plagiarism checker
Call for Paper
Volume 6 Issue 4
July-August 2024
Indexing Partners






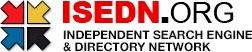

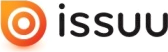


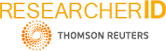
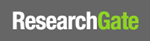
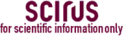
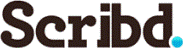

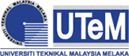
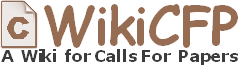
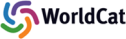
Location Based Privacy Preserving using Machine Learning
Author(s) | Roshini Singh, Guguloth Kiranmai, A. Sangeetha |
---|---|
Country | India |
Abstract | Location-based privacy preservation is crucial in the digital age due to the widespread use of location-based services and growing concerns about individual privacy. Despite the use of k-anonymity measures, current systems face challenges, particularly the risk of re-identification, especially when attackers have additional contextual information. These systems also suffer from information loss, leading to a significant decrease in data utility. Striking the right balance between privacy and data utility remains a prominent challenge in the field of location-based privacy preservation. To address the existing gaps and challenges in privacy-preserving location data publishing, a robust framework termed Location anonymization framework is being introduced which consists of three methods Generalization, Sequence alignment and Clustering. DGH Trees are being used to minimize the information loss and also maintains a balance between privacy and utility. To enhance privacy preservation in overly sensitive datasets, a modified version of the k-means algorithm is being put forth. Moreover, to improve the alignment process, the more efficient iterative multisequence alignment is being opted for over the progressive counterpart within this framework. The aim is to achieve a more balanced trade-off between privacy and utility in location data publishing. |
Keywords | Machine Learning,Domain Generalization Hierarchy, Location Based Privacy Preserving, Alignment, Clustering, Location Anonymization Framework, Data Privacy, Quasi Identifiers, Information Loss, Data Security, Privacy Trade-off metric, Pairwise Alignment of Residue Patterns. |
Field | Computer > Network / Security |
Published In | Volume 6, Issue 2, March-April 2024 |
Published On | 2024-04-30 |
Cite This | Location Based Privacy Preserving using Machine Learning - Roshini Singh, Guguloth Kiranmai, A. Sangeetha - IJFMR Volume 6, Issue 2, March-April 2024. DOI 10.36948/ijfmr.2024.v06i02.18868 |
DOI | https://doi.org/10.36948/ijfmr.2024.v06i02.18868 |
Short DOI | https://doi.org/gtsns7 |
Share this
E-ISSN 2582-2160

CrossRef DOI is assigned to each research paper published in our journal.
IJFMR DOI prefix is
10.36948/ijfmr
Downloads
All research papers published on this website are licensed under Creative Commons Attribution-ShareAlike 4.0 International License, and all rights belong to their respective authors/researchers.
