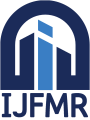
International Journal For Multidisciplinary Research
E-ISSN: 2582-2160
•
Impact Factor: 9.24
A Widely Indexed Open Access Peer Reviewed Multidisciplinary Bi-monthly Scholarly International Journal
Home
Research Paper
Submit Research Paper
Publication Guidelines
Publication Charges
Upload Documents
Track Status / Pay Fees / Download Publication Certi.
Editors & Reviewers
View All
Join as a Reviewer
Get Membership Certificate
Current Issue
Publication Archive
Conference
Publishing Conf. with IJFMR
Upcoming Conference(s) ↓
WSMCDD-2025
GSMCDD-2025
Conferences Published ↓
RBS:RH-COVID-19 (2023)
ICMRS'23
PIPRDA-2023
Contact Us
Plagiarism is checked by the leading plagiarism checker
Call for Paper
Volume 7 Issue 2
March-April 2025
Indexing Partners






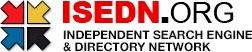

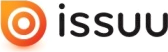


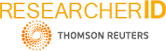
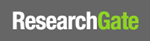
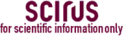
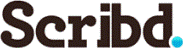

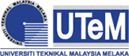
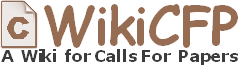
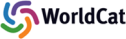
Predicting Student Placement using Machine Learning Models: A Comparative Analysis
Author(s) | Sumedh Tardalkar, Atharva Kale, Suyash Bhavsar, Varsha Shukre |
---|---|
Country | India |
Abstract | The transition from academia to the workforce marks a critical juncture for students, with the ability to predict their placement success becoming increasingly vital. This paper undertook a thorough comparative analysis of machine learning (ML) models applied in forecasting student placement. It delves into a range of factors influencing placement outcomes, encompassing academic performance, internship engagements, and demographic variables. Through an examination of the effectiveness of ML algorithms such as logistic regression, decision trees, random forests, and support vector machines, this study assesses their accuracy and efficacy in predicting student placements. The insights garnered from this analysis underscore the significance of internship experiences and academic achievements in shaping placement trajectories. Moreover, the research illuminates the crucial role of model selection and hyperparameter tuning in bolstering predictive capabilities. The findings gleaned from this study offer valuable insights into the intricate dynamics of student placement prediction, thereby aiding in the development of more precise and reliable ML models to assist students and educational institutions in navigating the multifaceted landscape of placement prediction. Throughout conducting this analysis Random Forest was found to be the most suitable prediction algorithm with over 81.47% accuracy in prediction placed and unplaced students. The dataset used had 2966 records which were collected from kaggle and various other sources or manually collected and converted into an csv file for conducting this analysis. |
Keywords | Supervised Learning, Unsupervised Learning, Bagging, Boosting, Placement Prediction |
Field | Computer > Artificial Intelligence / Simulation / Virtual Reality |
Published In | Volume 6, Issue 3, May-June 2024 |
Published On | 2024-05-31 |
Cite This | Predicting Student Placement using Machine Learning Models: A Comparative Analysis - Sumedh Tardalkar, Atharva Kale, Suyash Bhavsar, Varsha Shukre - IJFMR Volume 6, Issue 3, May-June 2024. DOI 10.36948/ijfmr.2024.v06i03.20340 |
DOI | https://doi.org/10.36948/ijfmr.2024.v06i03.20340 |
Short DOI | https://doi.org/gtw6tg |
Share this

E-ISSN 2582-2160

CrossRef DOI is assigned to each research paper published in our journal.
IJFMR DOI prefix is
10.36948/ijfmr
Downloads
All research papers published on this website are licensed under Creative Commons Attribution-ShareAlike 4.0 International License, and all rights belong to their respective authors/researchers.
