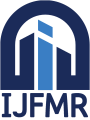
International Journal For Multidisciplinary Research
E-ISSN: 2582-2160
•
Impact Factor: 9.24
A Widely Indexed Open Access Peer Reviewed Multidisciplinary Bi-monthly Scholarly International Journal
Home
Research Paper
Submit Research Paper
Publication Guidelines
Publication Charges
Upload Documents
Track Status / Pay Fees / Download Publication Certi.
Editors & Reviewers
View All
Join as a Reviewer
Get Membership Certificate
Current Issue
Publication Archive
Conference
Publishing Conf. with IJFMR
Upcoming Conference(s) ↓
WSMCDD-2025
GSMCDD-2025
Conferences Published ↓
RBS:RH-COVID-19 (2023)
ICMRS'23
PIPRDA-2023
Contact Us
Plagiarism is checked by the leading plagiarism checker
Call for Paper
Volume 7 Issue 2
March-April 2025
Indexing Partners






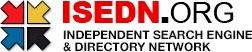

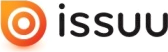


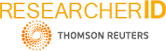
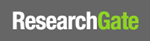
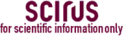
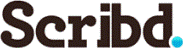

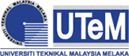
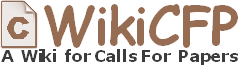
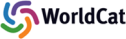
Fatigue State Detection for Tired Person in Presence of Driving Periods
Author(s) | S. Selvakani, K. Vasumathi, M. Jayaram |
---|---|
Country | India |
Abstract | Due to the increasing of traffic accidents, there is an urgent need to control and reduce driving mistakes. Driver fatigue or drowsiness is one of these major mistakes. Algorithms have been developed to address this issue by detecting fatigue and alerting the driver to dangerous condition. The major problem of the developed algorithms is their accuracy, well as the time required to detect fatigue status and alert the driver. The accuracy and time represent a critical condition that affects the reduction of traffic accidents. Several datasets have been used in the development of fatigue or drowsy detection techniques. These data gathered from the deriver's brain Electroencephalogram (EEG) signals or from video streaming recordings of the driver behavior. This paper develops two distinct approaches, the first based on the use of machine learning classifiers and the second depends on the use deep learning models to produce a high-performance fatigue detection system. The machine learning approach is used to process EEG signals, whereas the deep learning approach is used to process video streams. In machine learning classifiers, Support Vector Machine (SVM) provides up to 98% of detection accuracy, which is the highest accuracy among the other five deployed classifiers. In deep learning models, Convolution Neural Network (CNN) provides up to 99% detection accuracy, which is the highest accuracy among the other two deployed models. The experimental results demonstrate that the two proposed algorithms provide the highest detection accuracy with the shortest Testing Time (TT) when compared to all other recent and efficient fatigue detection algorithms. |
Keywords | EEG Signals, Fatigue Detection Systems, Support Vector Machine, Convolution Neural Network |
Field | Computer Applications |
Published In | Volume 5, Issue 2, March-April 2023 |
Published On | 2023-04-05 |
DOI | https://doi.org/10.36948/ijfmr.2023.v05i02.2056 |
Short DOI | https://doi.org/gr4k6h |
Share this

E-ISSN 2582-2160

CrossRef DOI is assigned to each research paper published in our journal.
IJFMR DOI prefix is
10.36948/ijfmr
Downloads
All research papers published on this website are licensed under Creative Commons Attribution-ShareAlike 4.0 International License, and all rights belong to their respective authors/researchers.
