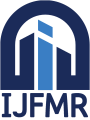
International Journal For Multidisciplinary Research
E-ISSN: 2582-2160
•
Impact Factor: 9.24
A Widely Indexed Open Access Peer Reviewed Multidisciplinary Bi-monthly Scholarly International Journal
Home
Research Paper
Submit Research Paper
Publication Guidelines
Publication Charges
Upload Documents
Track Status / Pay Fees / Download Publication Certi.
Editors & Reviewers
View All
Join as a Reviewer
Get Membership Certificate
Current Issue
Publication Archive
Conference
Publishing Conf. with IJFMR
Upcoming Conference(s) ↓
WSMCDD-2025
GSMCDD-2025
Conferences Published ↓
RBS:RH-COVID-19 (2023)
ICMRS'23
PIPRDA-2023
Contact Us
Plagiarism is checked by the leading plagiarism checker
Call for Paper
Volume 7 Issue 2
March-April 2025
Indexing Partners






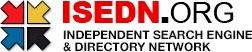

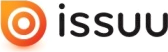


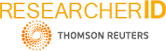
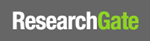
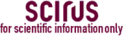
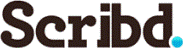

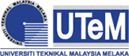
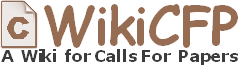
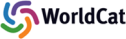
Dynamic BdSL Digit Recognition using Hybrid 3DCNN+BiLSTM Model
Author(s) | Ishat Salsabil Silvia, Kamrul Hasan Talukder |
---|---|
Country | Bangladesh |
Abstract | Sign language is used as a way of communication by deaf and mute individuals. However, due to the limited number of people who understand sign language, integrating them into society is challenging. Approximately 6.9% of Bangladesh's population and 5% of the world’s population suffer from speech impediments. Individuals with this condition cannot hear what others are saying or communicate verbally, thus sign language must be relied upon. In recent years, sign language recognition has gained attention due to its necessity. However, a scarcity of publicly available dynamic gesture datasets for Bangladeshi Sign Language (BdSL) exists. Dynamic gestures, which contain both spatial and temporal information, are more useful in real-life applications. The classification of dynamic gestures requires more data than static gestures. In this research, 11 numeral Bengali digit signs are aimed to be classified. Data has been collected from the “SignBD-Word” dataset, which contains extracted RGB human body pose keypoints skeleton data. When compared to other dynamic sign language datasets and data requirements for training deep neural networks for dynamic gestures, the data per class in this dataset is found to be insufficient. A hybrid model architecture is proposed in this research to recognize dynamic gestures using lightweight 3DCNN and bidirectional LSTM layers for classifying gestures from the motion patterns of human body pose keypoints skeleton data after experimenting on various models. It has been observed that combining 3DCNN with the pre-trained DenseNet-201 and the BiLSTM model increases real-time accuracy by 4.54%. To the best of our knowledge, this is the first approach to combine 3DCNN with DenseNet-201 for action recognition. Also, one of the earliest investigations on dynamic BdSL hand gesture digit recognition using dynamic data. Additionally, different pre-trained models as base feature extractors have been evaluated. |
Keywords | BdSL, Sign language, Deep learning, Transfer learning, Body joints skeleton, Action recognition |
Field | Computer > Artificial Intelligence / Simulation / Virtual Reality |
Published In | Volume 6, Issue 3, May-June 2024 |
Published On | 2024-06-17 |
Cite This | Dynamic BdSL Digit Recognition using Hybrid 3DCNN+BiLSTM Model - Ishat Salsabil Silvia, Kamrul Hasan Talukder - IJFMR Volume 6, Issue 3, May-June 2024. DOI 10.36948/ijfmr.2024.v06i03.20993 |
DOI | https://doi.org/10.36948/ijfmr.2024.v06i03.20993 |
Short DOI | https://doi.org/gt2cb3 |
Share this

E-ISSN 2582-2160

CrossRef DOI is assigned to each research paper published in our journal.
IJFMR DOI prefix is
10.36948/ijfmr
Downloads
All research papers published on this website are licensed under Creative Commons Attribution-ShareAlike 4.0 International License, and all rights belong to their respective authors/researchers.
