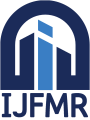
International Journal For Multidisciplinary Research
E-ISSN: 2582-2160
•
Impact Factor: 9.24
A Widely Indexed Open Access Peer Reviewed Multidisciplinary Bi-monthly Scholarly International Journal
Home
Research Paper
Submit Research Paper
Publication Guidelines
Publication Charges
Upload Documents
Track Status / Pay Fees / Download Publication Certi.
Editors & Reviewers
View All
Join as a Reviewer
Reviewer Referral Program
Get Membership Certificate
Current Issue
Publication Archive
Conference
Publishing Conf. with IJFMR
Upcoming Conference(s) ↓
WSMCDD-2025
GSMCDD-2025
Conferences Published ↓
RBS:RH-COVID-19 (2023)
ICMRS'23
PIPRDA-2023
Contact Us
Plagiarism is checked by the leading plagiarism checker
Call for Paper
Volume 6 Issue 6
November-December 2024
Indexing Partners






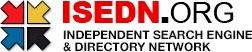

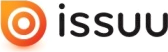


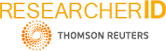
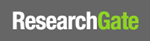
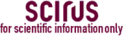
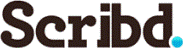

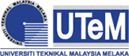
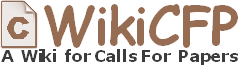
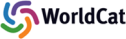
Enhanced Robotic Grasp Detection through Advanced Resnet Variants in Deep Convolutional Neural Networks
Author(s) | Tanya Thukral |
---|---|
Country | India |
Abstract | The advent of deep learning has propelled significant advancements in the field of robotic manipulation, enabling robots to perform complex grasping tasks with unprecedented accuracy. This study introduces an innovative approach to robotic grasp detection leveraging advanced variants of the Residual Network (ResNet) architecture within Deep Convolutional Neural Networks (DCNNs). Our methodology extends the conventional ResNet framework by integrating novel structural modifications and optimization techniques aimed at enhancing feature representation and learning efficiency. By incorporating dilated convolutions, attention mechanisms, and depth-wise separable convolutions, our proposed ResNet variants significantly improve the grasp detection performance in terms of accuracy, speed, and robustness across diverse object categories and orientations. The effectiveness of our approach is validated through extensive experiments on standard robotic grasp datasets, where our models demonstrate superior performance compared to existing state-of-the-art grasp detection methodologies. Furthermore, our analysis reveals that the advanced ResNet variants are capable of learning more discriminative features from grasp imagery, facilitating more precise localization and orientation prediction for robotic grippers. Additionally, the computational efficiency of our models enables real-time grasp detection, which is critical for practical robotic applications. Advanced techniques in deep learning have significantly propelled the fields of computer vision and natural language processing forward. Despite notable achievements, the integration of deep learning into robotics applications remains relatively limited. This study introduces a novel approach to robotic grasp detection, focusing on predicting the optimal grasping pose for a parallel-plate robotic gripper when encountering unfamiliar objects, utilizing RGB-D imagery of the scene. |
Field | Computer > Artificial Intelligence / Simulation / Virtual Reality |
Published In | Volume 6, Issue 4, July-August 2024 |
Published On | 2024-07-18 |
Cite This | Enhanced Robotic Grasp Detection through Advanced Resnet Variants in Deep Convolutional Neural Networks - Tanya Thukral - IJFMR Volume 6, Issue 4, July-August 2024. DOI 10.36948/ijfmr.2024.v06i04.21289 |
DOI | https://doi.org/10.36948/ijfmr.2024.v06i04.21289 |
Short DOI | https://doi.org/gt43xj |
Share this

E-ISSN 2582-2160

CrossRef DOI is assigned to each research paper published in our journal.
IJFMR DOI prefix is
10.36948/ijfmr
Downloads
All research papers published on this website are licensed under Creative Commons Attribution-ShareAlike 4.0 International License, and all rights belong to their respective authors/researchers.
