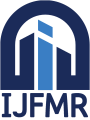
International Journal For Multidisciplinary Research
E-ISSN: 2582-2160
•
Impact Factor: 9.24
A Widely Indexed Open Access Peer Reviewed Multidisciplinary Bi-monthly Scholarly International Journal
Home
Research Paper
Submit Research Paper
Publication Guidelines
Publication Charges
Upload Documents
Track Status / Pay Fees / Download Publication Certi.
Editors & Reviewers
View All
Join as a Reviewer
Reviewer Referral Program
Get Membership Certificate
Current Issue
Publication Archive
Conference
Publishing Conf. with IJFMR
Upcoming Conference(s) ↓
WSMCDD-2025
Conferences Published ↓
RBS:RH-COVID-19 (2023)
ICMRS'23
PIPRDA-2023
Contact Us
Plagiarism is checked by the leading plagiarism checker
Call for Paper
Volume 6 Issue 4
July-August 2024
Indexing Partners






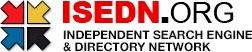

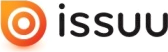


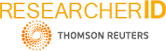
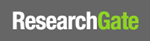
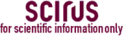
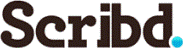

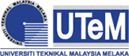
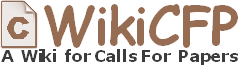
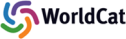
Detection Of phishing URLs using a Term Frequency Inverse Document Frequency (TF-IDF).
Author(s) | Sibhathallah M, Sathya Srinivas D |
---|---|
Country | India |
Abstract | Phishing attacks continue to pose a threat to online security as hackers employ more sophisticated tactics to trick users into revealing sensitive information. One common method is creating fake login URLs that mimic legitimate websites, making it challenging for users to distinguish between safe and dangerous links. This study focuses on detecting real-world phishing URLs, particularly analyzing login URLs. Our aim is to develop effective techniques and tools for early detection and prevention of phishing attempts by examining the common traits and patterns associated with them. The paper seeks to enhance people's and organizations' resilience to phishing attacks by exploring advanced technology and machine learning algorithms, ultimately contributing to a safer online environment. The research evaluates the effectiveness of deep learning and machine learning techniques in classifying phishing URLs, using statistical features such as Term Frequency-Inverse Document Frequency (TF-IDF) in combination with character N-gram, as well as proposed handcrafted features. CNN models are utilized for the deep learning approaches. Following model training, it is used to classify phishing URLs. The primary aim of this experiment is to assess the effectiveness of our proposed method. The results demonstrate that our phishing URL detection method achieves an accuracy rate of 96.6% |
Keywords | Cybercrime, Machine Learning, URLs, Prediction, Phishing Detection. |
Field | Computer > Artificial Intelligence / Simulation / Virtual Reality |
Published In | Volume 6, Issue 3, May-June 2024 |
Published On | 2024-06-01 |
Cite This | Detection Of phishing URLs using a Term Frequency Inverse Document Frequency (TF-IDF). - Sibhathallah M, Sathya Srinivas D - IJFMR Volume 6, Issue 3, May-June 2024. DOI 10.36948/ijfmr.2024.v06i03.21435 |
DOI | https://doi.org/10.36948/ijfmr.2024.v06i03.21435 |
Short DOI | https://doi.org/gtw6rt |
Share this

E-ISSN 2582-2160

CrossRef DOI is assigned to each research paper published in our journal.
IJFMR DOI prefix is
10.36948/ijfmr
Downloads
All research papers published on this website are licensed under Creative Commons Attribution-ShareAlike 4.0 International License, and all rights belong to their respective authors/researchers.
