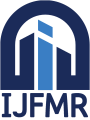
International Journal For Multidisciplinary Research
E-ISSN: 2582-2160
•
Impact Factor: 9.24
A Widely Indexed Open Access Peer Reviewed Multidisciplinary Bi-monthly Scholarly International Journal
Home
Research Paper
Submit Research Paper
Publication Guidelines
Publication Charges
Upload Documents
Track Status / Pay Fees / Download Publication Certi.
Editors & Reviewers
View All
Join as a Reviewer
Reviewer Referral Program
Get Membership Certificate
Current Issue
Publication Archive
Conference
Publishing Conf. with IJFMR
Upcoming Conference(s) ↓
WSMCDD-2025
Conferences Published ↓
RBS:RH-COVID-19 (2023)
ICMRS'23
PIPRDA-2023
Contact Us
Plagiarism is checked by the leading plagiarism checker
Call for Paper
Volume 6 Issue 4
July-August 2024
Indexing Partners






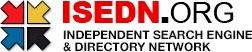

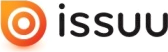


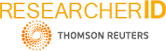
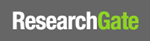
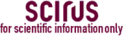
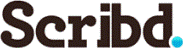

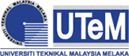
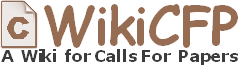
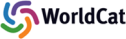
A Comparative Study of YOLO Models For Pneumonia Detection
Author(s) | Mohammed Saifuddin Munna, Rahul Chowdhury, Asif Mohammed Siddiqee |
---|---|
Country | Bangladesh |
Abstract | Traditional pneumonia detection methods are usually based on chest X-rays processed with the help of experienced radiologists, allowing for slow processing times and considerable bias. It can have the greatest of implications on the outcome of pneumonia that is a leading killer of children when its timely diagnosis is essential for proper treatment. For accelerated and automated pneumonia identification, deep learning is a prospective technique. Even though different techniques are suggested, some studies proved object detection models to be promising for disguise detection. In this study, we compared three YOLO models (YOLOv3, YOLOv4, and YOLOv6) to determine their performances in detecting pneumonia. We use a dataset of three-class chest X-rays for which we are asked to categorize chest X-rays into normal, viral pneumonia, and bacterial pneumonia. Here, we investigate the performance of different YOLO models to detect pneumonia and classify the type of pneumonia. We aim to demonstrate that the results show the superiority of YOLOv6 over YOLOv3 and YOLOv4, which may help speed up and improve the pneumonia identification in the clinic, ultimately supporting for early intervention and better patient prognosis. |
Keywords | Pneumonia, YOLOv3, YOLOv4, Deep Learning, YOLOv6 |
Field | Engineering |
Published In | Volume 6, Issue 3, May-June 2024 |
Published On | 2024-06-17 |
Cite This | A Comparative Study of YOLO Models For Pneumonia Detection - Mohammed Saifuddin Munna, Rahul Chowdhury, Asif Mohammed Siddiqee - IJFMR Volume 6, Issue 3, May-June 2024. DOI 10.36948/ijfmr.2024.v06i03.22770 |
DOI | https://doi.org/10.36948/ijfmr.2024.v06i03.22770 |
Short DOI | https://doi.org/gt2b8k |
Share this

E-ISSN 2582-2160

CrossRef DOI is assigned to each research paper published in our journal.
IJFMR DOI prefix is
10.36948/ijfmr
Downloads
All research papers published on this website are licensed under Creative Commons Attribution-ShareAlike 4.0 International License, and all rights belong to their respective authors/researchers.
