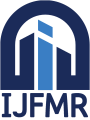
International Journal For Multidisciplinary Research
E-ISSN: 2582-2160
•
Impact Factor: 9.24
A Widely Indexed Open Access Peer Reviewed Multidisciplinary Bi-monthly Scholarly International Journal
Home
Research Paper
Submit Research Paper
Publication Guidelines
Publication Charges
Upload Documents
Track Status / Pay Fees / Download Publication Certi.
Editors & Reviewers
View All
Join as a Reviewer
Get Membership Certificate
Current Issue
Publication Archive
Conference
Publishing Conf. with IJFMR
Upcoming Conference(s) ↓
WSMCDD-2025
GSMCDD-2025
Conferences Published ↓
RBS:RH-COVID-19 (2023)
ICMRS'23
PIPRDA-2023
Contact Us
Plagiarism is checked by the leading plagiarism checker
Call for Paper
Volume 7 Issue 2
March-April 2025
Indexing Partners






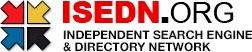

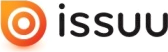


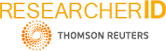
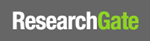
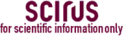
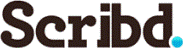

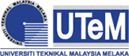
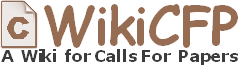
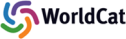
Enhancing Security and Privacy in Large Language Model-based Approaches: A Comprehensive Investigation
Author(s) | Pratyush Singhal |
---|---|
Country | India |
Abstract | During the last five years, there have been significant developments in the field of Natural Language Processing including the deployment of advanced large language models such as ChatGPT, Bard and Llama. These large language models are helpful in generating text and designing content and they have several applications in various industries. However, they can memorize and reveal malicious content and personal information from their training dataset which also includes an enormous amount of data from the internet. As a result, it can lead to compromised privacy and security challenges for users who have their personal information available on the internet directly or through third parties. To address this issue, the proposed research work conducts a thorough investigation of these challenges and puts forward a prompt designing-based solution. In this method, we build a customized training dataset to fine-tune a pre-trained model (Llama-2) to produce a harmless response ‘I can’t provide you with this information’ to prompts seeking to extract personal information and malicious content from LLMs. Experimental results reveal that the proposed work achieves an accuracy of 63% with a precision score of 0.706 and a recall score of 0.571. The work ensures almost no leakage of private information and strengthens the LLM model against extraction attacks. |
Keywords | Deep learning, Prompt designing, Large Language Models |
Field | Computer > Data / Information |
Published In | Volume 6, Issue 4, July-August 2024 |
Published On | 2024-07-12 |
DOI | https://doi.org/10.36948/ijfmr.2024.v06i04.24476 |
Short DOI | https://doi.org/gt4gjm |
Share this

E-ISSN 2582-2160

CrossRef DOI is assigned to each research paper published in our journal.
IJFMR DOI prefix is
10.36948/ijfmr
Downloads
All research papers published on this website are licensed under Creative Commons Attribution-ShareAlike 4.0 International License, and all rights belong to their respective authors/researchers.
