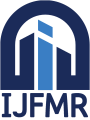
International Journal For Multidisciplinary Research
E-ISSN: 2582-2160
•
Impact Factor: 9.24
A Widely Indexed Open Access Peer Reviewed Multidisciplinary Bi-monthly Scholarly International Journal
Home
Research Paper
Submit Research Paper
Publication Guidelines
Publication Charges
Upload Documents
Track Status / Pay Fees / Download Publication Certi.
Editors & Reviewers
View All
Join as a Reviewer
Get Membership Certificate
Current Issue
Publication Archive
Conference
Publishing Conf. with IJFMR
Upcoming Conference(s) ↓
WSMCDD-2025
GSMCDD-2025
Conferences Published ↓
RBS:RH-COVID-19 (2023)
ICMRS'23
PIPRDA-2023
Contact Us
Plagiarism is checked by the leading plagiarism checker
Call for Paper
Volume 7 Issue 2
March-April 2025
Indexing Partners






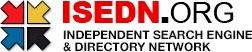

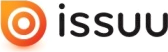


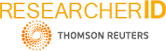
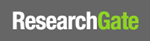
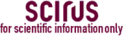
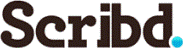

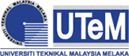
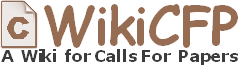
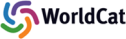
A comparative Study on AI-Driven Anonymization Techniques for Protecting Personal Data
Author(s) | Mahendralal Prajapati, Alok Kumar Upadhyay, Mehdi Rezaie, Jyotshna Dongradive |
---|---|
Country | India |
Abstract | In the Artificial Intelligence era, protecting individual users' data has become crucial. The collected data is stored in multiple databases having personally identifiable information (PII). This may provide a significant privacy concern for the database. Several privacy-preserving approaches have been proposed, including Differential Privacy, Homomorphic Encryption, Generative Adversarial Network and Federated Learning. In this paper, the above four anonymization techniques are compared. In addition, this study will review the strengths and weaknesses of these techniques. We also discuss the trade-off between data utility and privacy. The results of this study aim to guide researchers and practitioners in selecting suitable AI-driven anonymization techniques. |
Keywords | AI-driven data Anonymization, Data privacy, Differential privacy, literature review, homomorphic encryption, Generative Adversarial Networks, Federated Learning |
Field | Computer > Data / Information |
Published In | Volume 6, Issue 4, July-August 2024 |
Published On | 2024-07-18 |
DOI | https://doi.org/10.36948/ijfmr.2024.v06i04.24770 |
Short DOI | https://doi.org/gt43vs |
Share this

E-ISSN 2582-2160

CrossRef DOI is assigned to each research paper published in our journal.
IJFMR DOI prefix is
10.36948/ijfmr
Downloads
All research papers published on this website are licensed under Creative Commons Attribution-ShareAlike 4.0 International License, and all rights belong to their respective authors/researchers.
