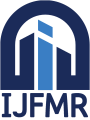
International Journal For Multidisciplinary Research
E-ISSN: 2582-2160
•
Impact Factor: 9.24
A Widely Indexed Open Access Peer Reviewed Multidisciplinary Bi-monthly Scholarly International Journal
Home
Research Paper
Submit Research Paper
Publication Guidelines
Publication Charges
Upload Documents
Track Status / Pay Fees / Download Publication Certi.
Editors & Reviewers
View All
Join as a Reviewer
Reviewer Referral Program
Get Membership Certificate
Current Issue
Publication Archive
Conference
Publishing Conf. with IJFMR
Upcoming Conference(s) ↓
WSMCDD-2025
GSMCDD-2025
Conferences Published ↓
RBS:RH-COVID-19 (2023)
ICMRS'23
PIPRDA-2023
Contact Us
Plagiarism is checked by the leading plagiarism checker
Call for Paper
Volume 6 Issue 6
November-December 2024
Indexing Partners






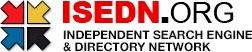

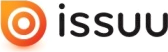


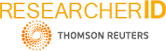
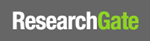
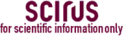
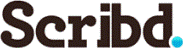

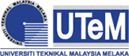
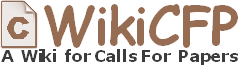
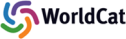
Predicting Job Change Intentions for a Food Manufacturing Company: A Deep Learning Case Study
Author(s) | Rachid Ejjami |
---|---|
Country | France |
Abstract | This study aims to address employee job change intention in the competitive food manufacturing industry by developing deep learning models to predict job change intentions. Using a comprehensive dataset of 32,000 employee records, including demographics, education, and work experience, the study employed a quantitative methodology with neural network analysis. Various deep learning models were implemented and evaluated using TensorFlow and Keras, with techniques like GridSearchCV, Random Search CV, SMOTE, and Keras Tuner used for hyperparameter tuning and addressing class imbalance. The findings revealed significant differences in model effectiveness, with Model 7: Complex Neural Network Architecture, featuring a complex architecture and appropriate regularization, achieving a reasonable balance across metrics and demonstrating improved recall for job changers. This suggests its suitability for predicting job change intention in a food manufacturing company. The study concludes that well-tuned deep learning models can significantly enhance predictive accuracy, offering valuable insights for HR professionals to develop targeted retention strategies. Future research should explore additional features influencing staff job change intention, validate these models across diverse organizational contexts, and integrate real-time data analytics and explainable AI techniques to improve transparency and effectiveness in HR practices. |
Keywords | Employee job change intention, Deep learning models, Food manufacturing industry, Neural network analysis, Hyperparameter tuning, SMOTE, Keras tuner, Workforce retention strategies, HR analytics, Predictive analytics, Employee engagement, Class imbalance, Data-driven HR, Talent management. |
Published In | Volume 6, Issue 4, July-August 2024 |
Published On | 2024-07-20 |
Cite This | Predicting Job Change Intentions for a Food Manufacturing Company: A Deep Learning Case Study - Rachid Ejjami - IJFMR Volume 6, Issue 4, July-August 2024. DOI 10.36948/ijfmr.2024.v06i04.24871 |
DOI | https://doi.org/10.36948/ijfmr.2024.v06i04.24871 |
Short DOI | https://doi.org/gt43td |
Share this

E-ISSN 2582-2160

CrossRef DOI is assigned to each research paper published in our journal.
IJFMR DOI prefix is
10.36948/ijfmr
Downloads
All research papers published on this website are licensed under Creative Commons Attribution-ShareAlike 4.0 International License, and all rights belong to their respective authors/researchers.
