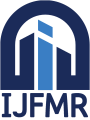
International Journal For Multidisciplinary Research
E-ISSN: 2582-2160
•
Impact Factor: 9.24
A Widely Indexed Open Access Peer Reviewed Multidisciplinary Bi-monthly Scholarly International Journal
Home
Research Paper
Submit Research Paper
Publication Guidelines
Publication Charges
Upload Documents
Track Status / Pay Fees / Download Publication Certi.
Editors & Reviewers
View All
Join as a Reviewer
Reviewer Referral Program
Get Membership Certificate
Current Issue
Publication Archive
Conference
Publishing Conf. with IJFMR
Upcoming Conference(s) ↓
WSMCDD-2025
GSMCDD-2025
Conferences Published ↓
RBS:RH-COVID-19 (2023)
ICMRS'23
PIPRDA-2023
Contact Us
Plagiarism is checked by the leading plagiarism checker
Call for Paper
Volume 6 Issue 6
November-December 2024
Indexing Partners






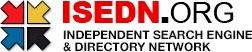

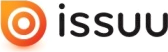


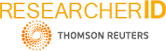
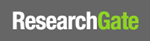
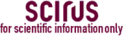
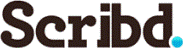

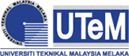
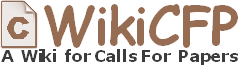
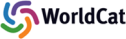
Hybrid approach for Recurrence of Cardiovascular Disease Prediction Using Machine Learning Techniques
Author(s) | A Manjesh gowda, Anish H G, Dr. Lakshmi K |
---|---|
Country | India |
Abstract | Cardiovascular disease remains a global health concern, being a leading cause of mortality. In response to the critical nature of heart-related conditions, this research focuses on the development of smart systems leveraging machine learning algorithms for accurate and timely diagnosis. The study explores various machine learning approaches to predict recurrence of heart diseases based on patient data encompassing key health factors. With heart disease being a prevalent cause of death worldwide, real-time forecasting methods from medical data sources have become crucial. The implementation of machine learning in healthcare demonstrates its potential for early and precise recurrence of disease detection. Despite the abundance of health information generated by medical institutions, there is an underutilization of this data, leading to a healthcare system that is "data rich" but "knowledge poor." This work aims to address this gap by presenting a reliable recurrence heart disease prediction system. The research highlights the necessity for effective analysis methods to uncover connections and patterns within healthcare data. The proposed prediction system utilizes a diverse set of health factors, contributing to a comprehensive understanding of heart disease. By leveraging machine learning algorithms, the system aims to enhance the accuracy and efficiency of diagnosis. The findings of this research not only contribute to the advancement of predictive healthcare but also underscore the significance of unlocking valuable insights from the vast pool of medical data. Ultimately, the implementation of such intelligent systems holds promise for improving patient outcomes and reducing the burden of cardiovascular diseases on global healthcare systems. The paper demonstrated Hybrid methods: Support Vector Machine (SVM), Random Forest (RF), and Naïve Bayes (NB), to build the prediction models. Data preprocessing and feature selection steps were done before building the models. The models were evaluated based on the accuracy, precision, recall, and F1-score. The Random Forest model performed best with 94.27% accuracy. |
Keywords | recurrence heart disease prediction, machine learning, hybrid approach, support vector machine, naïve bayes, random forest. |
Field | Computer > Data / Information |
Published In | Volume 6, Issue 4, July-August 2024 |
Published On | 2024-08-04 |
Cite This | Hybrid approach for Recurrence of Cardiovascular Disease Prediction Using Machine Learning Techniques - A Manjesh gowda, Anish H G, Dr. Lakshmi K - IJFMR Volume 6, Issue 4, July-August 2024. DOI 10.36948/ijfmr.2024.v06i04.25729 |
DOI | https://doi.org/10.36948/ijfmr.2024.v06i04.25729 |
Short DOI | https://doi.org/gt55w8 |
Share this

E-ISSN 2582-2160

CrossRef DOI is assigned to each research paper published in our journal.
IJFMR DOI prefix is
10.36948/ijfmr
Downloads
All research papers published on this website are licensed under Creative Commons Attribution-ShareAlike 4.0 International License, and all rights belong to their respective authors/researchers.
