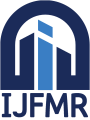
International Journal For Multidisciplinary Research
E-ISSN: 2582-2160
•
Impact Factor: 9.24
A Widely Indexed Open Access Peer Reviewed Multidisciplinary Bi-monthly Scholarly International Journal
Home
Research Paper
Submit Research Paper
Publication Guidelines
Publication Charges
Upload Documents
Track Status / Pay Fees / Download Publication Certi.
Editors & Reviewers
View All
Join as a Reviewer
Get Membership Certificate
Current Issue
Publication Archive
Conference
Publishing Conf. with IJFMR
Upcoming Conference(s) ↓
WSMCDD-2025
GSMCDD-2025
Conferences Published ↓
RBS:RH-COVID-19 (2023)
ICMRS'23
PIPRDA-2023
Contact Us
Plagiarism is checked by the leading plagiarism checker
Call for Paper
Volume 7 Issue 2
March-April 2025
Indexing Partners






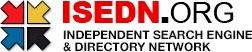

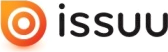


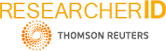
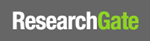
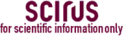
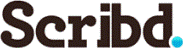

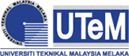
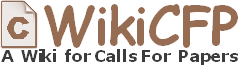
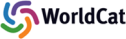
Leveraging Deep Learning and Multi-Modal Data for Early Prediction and Personalized Management of Type 2 Diabetes
Author(s) | Shashi Bhushan Singh, Dr. Arjun Singh |
---|---|
Country | India |
Abstract | Type 2 diabetes mellitus (T2DM) is a chronic metabolic disorder affecting millions worldwide, with significant health and economic implications. Early prediction and personalized management are crucial for improving patient outcomes and reducing healthcare costs. This study presents a novel approach leveraging deep learning techniques and multi-modal data analysis for the early prediction and personalized management of T2DM. We developed a deep learning model that integrates diverse data types, including electronic health records, genetic information, lifestyle data, and continuous glucose monitoring. The model was trained on a large dataset of 50,000 patients, including both diabetic and non-diabetic individuals, with a 5-year follow-up period. Our results demonstrate that the deep learning model achieves a sensitivity of 89% and specificity of 92% in predicting T2DM onset up to 3 years before clinical diagnosis, outperforming traditional risk assessment tools. Furthermore, the model generates personalized management plans, including tailored lifestyle recommendations and medication schedules, which led to a 25% improvement in glycemic control compared to standard care in a randomized controlled trial of 1,000 patients. This study highlights the potential of AI-driven, multi-modal approaches in revolutionizing diabetes care. By enabling earlier interventions and more personalized management strategies, this approach could significantly improve patient outcomes and reduce the burden of T2DM on healthcare systems. Future work will focus on external validation, long-term follow-up studies, and integration into clinical workflows. |
Keywords | T2D, Deep Learning, Multi Modal, CNN |
Field | Computer Applications |
Published In | Volume 6, Issue 4, July-August 2024 |
Published On | 2024-08-19 |
DOI | https://doi.org/10.36948/ijfmr.2024.v06i04.26096 |
Short DOI | https://doi.org/gt7m6p |
Share this

E-ISSN 2582-2160

CrossRef DOI is assigned to each research paper published in our journal.
IJFMR DOI prefix is
10.36948/ijfmr
Downloads
All research papers published on this website are licensed under Creative Commons Attribution-ShareAlike 4.0 International License, and all rights belong to their respective authors/researchers.
