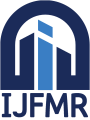
International Journal For Multidisciplinary Research
E-ISSN: 2582-2160
•
Impact Factor: 9.24
A Widely Indexed Open Access Peer Reviewed Multidisciplinary Bi-monthly Scholarly International Journal
Home
Research Paper
Submit Research Paper
Publication Guidelines
Publication Charges
Upload Documents
Track Status / Pay Fees / Download Publication Certi.
Editors & Reviewers
View All
Join as a Reviewer
Reviewer Referral Program
Get Membership Certificate
Current Issue
Publication Archive
Conference
Publishing Conf. with IJFMR
Upcoming Conference(s) ↓
WSMCDD-2025
Conferences Published ↓
RBS:RH-COVID-19 (2023)
ICMRS'23
PIPRDA-2023
Contact Us
Plagiarism is checked by the leading plagiarism checker
Call for Paper
Volume 6 Issue 4
July-August 2024
Indexing Partners






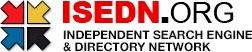

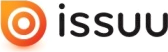


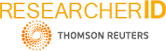
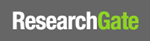
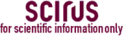
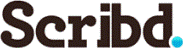

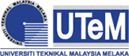
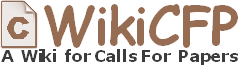
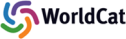
Heart Disease Risk Assessment by Using Light GBM Technique
Author(s) | N.M.K Ramalingamsakthivelan, V. Silambarasan, S. Thavasi, P. Vijaya Shankar |
---|---|
Country | India |
Abstract | Heart disease is a term that covers various illnesses affecting the heart, which may involve blood vessels, heart rhythm, or congenital abnormalities. The World Health Organization (WHO) has identified heart disease as one of the primary causes of death globally. Heart disease includes conditions such as coronary artery disease and arrhythmias, among others. Predicting Heart disease risk can help doctors accurately assess patients' health, allowing for early intervention and lifestyle changes or medical treatment as needed. Machine learning (ML) can aid in understanding and reducing symptoms of heart disease by using key parameters such as heart rate, body temperature, and blood pressure. To more accurately predict heart disease risk and alert medical professionals and caregivers to patient location, the Light Gradient Boosting Machine (LightGBM) technique is proposed. LightGBM modelling is a promising classification strategy for predicting medication adherence in heart disease patients, assisting with patient stratification and decision-making based on the best available data. The chi-square statistical test is utilized to select specific features from the Cleveland heart disease (HD) dataset, and data visualization is used to depict feature relationships. In 303 instances of Cleveland HD dataset attributes, the random forest algorithm achieved an 88.5% accuracy rate during validation, according to experiment results. |
Keywords | Keywords: Heart disease, Machine learning, Light Gradient Boosting Machine (LightGBM)technique, chi-square statistical test. |
Field | Computer > Artificial Intelligence / Simulation / Virtual Reality |
Published In | Volume 5, Issue 2, March-April 2023 |
Published On | 2023-04-26 |
Cite This | Heart Disease Risk Assessment by Using Light GBM Technique - N.M.K Ramalingamsakthivelan, V. Silambarasan, S. Thavasi, P. Vijaya Shankar - IJFMR Volume 5, Issue 2, March-April 2023. DOI 10.36948/ijfmr.2023.v05i02.2620 |
DOI | https://doi.org/10.36948/ijfmr.2023.v05i02.2620 |
Short DOI | https://doi.org/gr6h7b |
Share this
E-ISSN 2582-2160

CrossRef DOI is assigned to each research paper published in our journal.
IJFMR DOI prefix is
10.36948/ijfmr
Downloads
All research papers published on this website are licensed under Creative Commons Attribution-ShareAlike 4.0 International License, and all rights belong to their respective authors/researchers.
