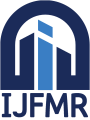
International Journal For Multidisciplinary Research
E-ISSN: 2582-2160
•
Impact Factor: 9.24
A Widely Indexed Open Access Peer Reviewed Multidisciplinary Bi-monthly Scholarly International Journal
Home
Research Paper
Submit Research Paper
Publication Guidelines
Publication Charges
Upload Documents
Track Status / Pay Fees / Download Publication Certi.
Editors & Reviewers
View All
Join as a Reviewer
Reviewer Referral Program
Get Membership Certificate
Current Issue
Publication Archive
Conference
Publishing Conf. with IJFMR
Upcoming Conference(s) ↓
WSMCDD-2025
GSMCDD-2025
Conferences Published ↓
RBS:RH-COVID-19 (2023)
ICMRS'23
PIPRDA-2023
Contact Us
Plagiarism is checked by the leading plagiarism checker
Call for Paper
Volume 6 Issue 6
November-December 2024
Indexing Partners






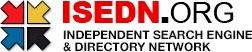

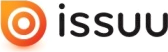


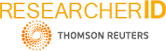
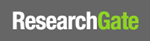
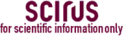
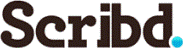

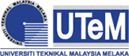
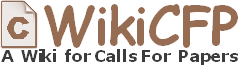
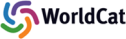
Detecting Intruders in Network using Machine Learning
Author(s) | Sujith.S, S.Uma.M.E.,, R.Sharath, K.Vasanth |
---|---|
Country | India |
Abstract | Malware detection plays a crucial role in cyber-security with the increase in malware growth and advancements in cyber-attacks. Malicious software applications, or malware, are the primary source of many security problems. These intentionally manipulative malicious applications intend to perform unauthorized activities on behalf of their originators on the host machines for various reasons such as stealing advanced technologies and intellectual properties, governmental acts of revenge, and tampering sensitive information, to name a few. Malware detection methods rely on signature databases, including malicious instruction patterns in today's practice. The signature databases are used for matching against a signature generated from a newly encountered executable. Nevertheless, more efficient mitigation methods are needed due to the fast expansion of malicious software on the Internet and their self- modifying abilities, as in polymorphic and metamorphic malware. In this work, it detects Network Intrusion anomalies by using NSL-KDD dataset. The user enters the hacking parameters in the front end. The model predicts the type of attack and gives information about the type of attack to the user. The project is fully responsive and completely based on session and cookies (Client-server protocol). Then we activated our malware security device which forms production for the set of attack. This will help many cyber threads |
Keywords | Intruders detection, NSL-KDD dataset, Client- Server protocol. |
Field | Computer > Network / Security |
Published In | Volume 5, Issue 2, March-April 2023 |
Published On | 2023-04-26 |
Cite This | Detecting Intruders in Network using Machine Learning - Sujith.S, S.Uma.M.E.,, R.Sharath, K.Vasanth - IJFMR Volume 5, Issue 2, March-April 2023. DOI 10.36948/ijfmr.2023.v05i02.2637 |
DOI | https://doi.org/10.36948/ijfmr.2023.v05i02.2637 |
Short DOI | https://doi.org/gr6h62 |
Share this

E-ISSN 2582-2160

CrossRef DOI is assigned to each research paper published in our journal.
IJFMR DOI prefix is
10.36948/ijfmr
Downloads
All research papers published on this website are licensed under Creative Commons Attribution-ShareAlike 4.0 International License, and all rights belong to their respective authors/researchers.
