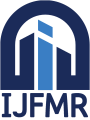
International Journal For Multidisciplinary Research
E-ISSN: 2582-2160
•
Impact Factor: 9.24
A Widely Indexed Open Access Peer Reviewed Multidisciplinary Bi-monthly Scholarly International Journal
Home
Research Paper
Submit Research Paper
Publication Guidelines
Publication Charges
Upload Documents
Track Status / Pay Fees / Download Publication Certi.
Editors & Reviewers
View All
Join as a Reviewer
Get Membership Certificate
Current Issue
Publication Archive
Conference
Publishing Conf. with IJFMR
Upcoming Conference(s) ↓
WSMCDD-2025
GSMCDD-2025
Conferences Published ↓
RBS:RH-COVID-19 (2023)
ICMRS'23
PIPRDA-2023
Contact Us
Plagiarism is checked by the leading plagiarism checker
Call for Paper
Volume 7 Issue 2
March-April 2025
Indexing Partners






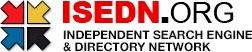

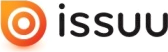


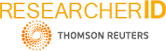
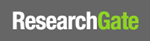
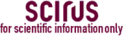
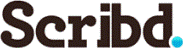

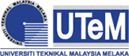
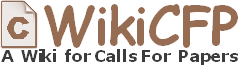
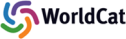
Anomalous Network Behaviour Detection in Interoperable Health Systems using Machine Learning in Resource Limited Areas
Author(s) | Marseline Michael Mtey, Anael Elikana Sam, Mussa Ally Dida |
---|---|
Country | Tanzania |
Abstract | The connection of devices in distributed environments produces and shares a vast amount of data useful for different organisational decision-making. In healthcare service organisations, for example, multiple e-health systems from different departments or facilities connect and share health data and information. During sharing, proper management is important to ensure the information is secure against intruders. Machine learning as a non-conventional security technique can be used along conventional techniques like firewalls, antivirus and intrusion detection systems to predict future network threats and other anomalies using historical backgrounds and other features. However, some machine learning algorithms have complex computation thus requiring resourceful systems in terms of network bandwidth, CPU power, memory, and storage capacity. In resource-constrained environments, therefore, special consideration is needed to ensure that the analysis of the big data is successful and that the benefits associated with them are effectively obtained. In this paper, a Machine Learning algorithm was selected among four algorithms whose performance was compared through various performance metrics. Classification accuracy, Mean Absolute Error (MAE), Root Mean Square Error (RMSE), and Relative Absolute Error (RAE) among other performance metrics were used to compare the ANN, Random forest, Decision trees, and Naïve byes classification algorithms using an extract from CICDDOS2019 dataset. Using the Weka version 3.8.6, the algorithms were compared to choose the best one to classify the data. By using three computers with different resources, the experiments were carried out to determine the performance of those machine learning algorithms. The result revealed that the random forest produced a good average classification performance in resource-limited systems since it surpassed other algorithms in classifying the data at an average of 99 per cent with a low average mean absolute error of 0.0001. Furthermore, as an ensemble that classifies with multiple decision trees algorithm, it likewise uses reasonable time to build and test the model therefore recommended for resource-limited systems. |
Keywords | Machine learning algorithms, Interoperability, Big data, Security, Anomalous behaviour, Resource limited areas, e-health system, Tanzania, Data Sharing |
Field | Computer > Network / Security |
Published In | Volume 6, Issue 4, July-August 2024 |
Published On | 2024-08-26 |
DOI | https://doi.org/10.36948/ijfmr.2024.v06i04.26455 |
Short DOI | https://doi.org/gt8g5b |
Share this

E-ISSN 2582-2160

CrossRef DOI is assigned to each research paper published in our journal.
IJFMR DOI prefix is
10.36948/ijfmr
Downloads
All research papers published on this website are licensed under Creative Commons Attribution-ShareAlike 4.0 International License, and all rights belong to their respective authors/researchers.
