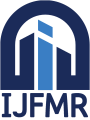
International Journal For Multidisciplinary Research
E-ISSN: 2582-2160
•
Impact Factor: 9.24
A Widely Indexed Open Access Peer Reviewed Multidisciplinary Bi-monthly Scholarly International Journal
Home
Research Paper
Submit Research Paper
Publication Guidelines
Publication Charges
Upload Documents
Track Status / Pay Fees / Download Publication Certi.
Editors & Reviewers
View All
Join as a Reviewer
Get Membership Certificate
Current Issue
Publication Archive
Conference
Publishing Conf. with IJFMR
Upcoming Conference(s) ↓
WSMCDD-2025
GSMCDD-2025
Conferences Published ↓
RBS:RH-COVID-19 (2023)
ICMRS'23
PIPRDA-2023
Contact Us
Plagiarism is checked by the leading plagiarism checker
Call for Paper
Volume 7 Issue 2
March-April 2025
Indexing Partners






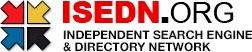

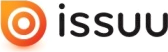


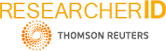
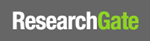
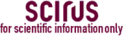
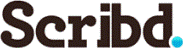

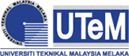
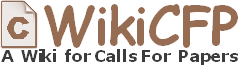
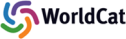
Estimating Time to Progression of Chronic Obstructive Pulmonary Disease with Tolerance
Author(s) | Vijayvenkateshwaran M., Murali T., Santhosh B., Sathish B. |
---|---|
Country | India |
Abstract | Chronic Obstructive Pulmonary Disease (COPD) is a progressive respiratory disease that affects millions of people worldwide. Predicting the time to progression of COPD is critical for optimizing patient care and treatment planning. However, COPD progression is complex and multifactorial, and estimating time to progression accurately remains challenging. In this study, we propose a novel approach for estimating time to progression of COPD using a Random Forest model. Random Forest is an ensemble machine learning technique that combines multiple decision trees to make predictions. We incorporated the concept of tolerance into our model, which allows for variability in COPD progression rates among individuals. We utilized a dataset of COPD patients, including clinical and demographic variables, collected over a multi-year period. The dataset was divided into training and testing sets for model development and evaluation, respectively. The Random Forest model was trained on the training set using features such as age, gender, smoking history, lung function parameters, and comorbidities. The Random Forest model with tolerance outperformed traditional models in estimating time to progression of COPD. The tolerance concept allowed the model to account for inter-individual variability in COPD progression rates, which is a critical aspect of COPD prognosis. The model achieved high accuracy and robustness in predicting time to progression of COPD in the testing set, indicating its potential clinical utility. A novel approach for estimating time to progression of COPD using a Random Forest model with tolerance. This approach has the potential to improve the accuracy of COPD prognosis, leading to better patient care and treatment planning. Further validation studies are warranted to validate the clinical utility of our proposed model in diverse COPD populations.This project describes the pulmonary chronic disease detection and classification is done with different observing similar disease conditions or functional status from the upper to the lower boundaries of a specified time interval Different types of radiological report is analyzed for different radiologic reports disease detection and classification is done with different radiological reports The proposed model is compared with different machine learning models compared with different stages of COPD Chronic Obstructive Pulmonary Disease |
Keywords | Random Forest, COPD Dataset, Radiological Report |
Field | Medical / Pharmacy |
Published In | Volume 5, Issue 2, March-April 2023 |
Published On | 2023-04-26 |
DOI | https://doi.org/10.36948/ijfmr.2023.v05i02.2673 |
Short DOI | https://doi.org/gr7hgd |
Share this

E-ISSN 2582-2160

CrossRef DOI is assigned to each research paper published in our journal.
IJFMR DOI prefix is
10.36948/ijfmr
Downloads
All research papers published on this website are licensed under Creative Commons Attribution-ShareAlike 4.0 International License, and all rights belong to their respective authors/researchers.
