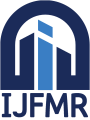
International Journal For Multidisciplinary Research
E-ISSN: 2582-2160
•
Impact Factor: 9.24
A Widely Indexed Open Access Peer Reviewed Multidisciplinary Bi-monthly Scholarly International Journal
Home
Research Paper
Submit Research Paper
Publication Guidelines
Publication Charges
Upload Documents
Track Status / Pay Fees / Download Publication Certi.
Editors & Reviewers
View All
Join as a Reviewer
Get Membership Certificate
Current Issue
Publication Archive
Conference
Publishing Conf. with IJFMR
Upcoming Conference(s) ↓
WSMCDD-2025
GSMCDD-2025
Conferences Published ↓
RBS:RH-COVID-19 (2023)
ICMRS'23
PIPRDA-2023
Contact Us
Plagiarism is checked by the leading plagiarism checker
Call for Paper
Volume 7 Issue 2
March-April 2025
Indexing Partners






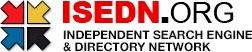

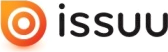


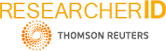
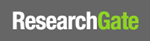
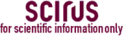
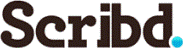

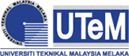
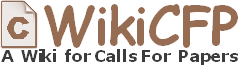
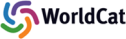
Research on Thyroid Cancer Detection and Classification Using Deep Learning over Ultrasound Images
Author(s) | Pavithra S, Naveen Kumar J.R |
---|---|
Country | India |
Abstract | The automated classification of thyroid nodules using ultrasound images is a crucial method for detecting thyroid nodules and improving diagnostic accuracy. This project aims to develop a novel model based on deep learning, specifically utilizing convolution neural network (CNNs). In the field of medical images analysis, the application of deep learning techniques has garnered significant attention for detecting and classifying thyroid cancer, particularly through the analysis of ultrasound images. This research investigates the potential of CNNs and other deep learning algorithm to enhance the precision and efficiency of thyroid cancer diagnosis. By processing benign and malignant thyroid nodules ultimately aiding in early and accurate detection, which is vital for better patient outcomes and informed technology with medical diagnostics, showcasing the significant advancements deep learning brings to the identification and classification of thyroid cancer using ultrasound imaging. |
Keywords | Convolution Neural Network (CNNs), Thyroid Cancer, Ultrasound Images, and Deep Learning. |
Field | Computer > Artificial Intelligence / Simulation / Virtual Reality |
Published In | Volume 6, Issue 4, July-August 2024 |
Published On | 2024-08-31 |
DOI | https://doi.org/10.36948/ijfmr.2024.v06i04.26749 |
Short DOI | https://doi.org/gt9hdm |
Share this

E-ISSN 2582-2160

CrossRef DOI is assigned to each research paper published in our journal.
IJFMR DOI prefix is
10.36948/ijfmr
Downloads
All research papers published on this website are licensed under Creative Commons Attribution-ShareAlike 4.0 International License, and all rights belong to their respective authors/researchers.
