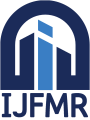
International Journal For Multidisciplinary Research
E-ISSN: 2582-2160
•
Impact Factor: 9.24
A Widely Indexed Open Access Peer Reviewed Multidisciplinary Bi-monthly Scholarly International Journal
Home
Research Paper
Submit Research Paper
Publication Guidelines
Publication Charges
Upload Documents
Track Status / Pay Fees / Download Publication Certi.
Editors & Reviewers
View All
Join as a Reviewer
Get Membership Certificate
Current Issue
Publication Archive
Conference
Publishing Conf. with IJFMR
Upcoming Conference(s) ↓
WSMCDD-2025
GSMCDD-2025
AIMAR-2025
Conferences Published ↓
ICCE (2025)
RBS:RH-COVID-19 (2023)
ICMRS'23
PIPRDA-2023
Contact Us
Plagiarism is checked by the leading plagiarism checker
Call for Paper
Volume 7 Issue 4
July-August 2025
Indexing Partners






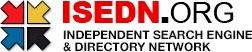

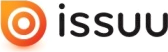


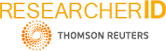
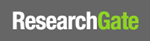
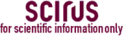
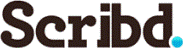

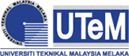
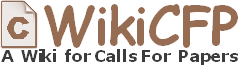
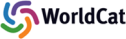
Comparative Evaluation of K-Means, Hierarchical Clustering, and DBSCAN in Blood Donor Segmentation
Author(s) | Srilekha S, Priyadharshini. P, Adhilakshmi. M |
---|---|
Country | India |
Abstract | Clustering techniques are pivotal in the fields of data analysis and pattern recognition, offering significant insights by grouping data points with similar characteristics. This study aims to perform a comprehensive comparison of three widely used clustering algorithms—K-Means, Hierarchical Clustering, and DBSCAN—on a dataset of blood donors. The objective is to determine which algorithm achieves the most precise and effective clustering of the data, taking into account factors such as donor location, blood type, and donation frequency. The study presents a novel approach by integrating a web-based platform that allows blood donors to register online. This platform not only facilitates the real-time updating of the dataset but also enhances the overall relevance and applicability of the clustering model by continuously incorporating new data entries. By leveraging such a dynamic dataset, the clustering algorithms can adapt to evolving patterns and trends, ensuring more accurate and meaningful insights over time.To rigorously evaluate the performance of each clustering method, several well-established metrics are employed, including the Silhouette Score, which assesses how similar each data point is to its own cluster compared to other clusters; the Davies-Bouldin Index, which evaluates the average similarity ratio of each cluster with its most similar cluster; and the Calinski-Harabasz Index, which measures the ratio of the sum of between-clusters dispersion and of within-cluster dispersion for all clusters. The results of this study indicate that the K-Means algorithm consistently outperforms both Hierarchical Clustering and DBSCAN in terms of accuracy and the clarity of cluster definitions. The findings underscore the robustness of K-Means for applications involving blood donor data, where capturing precise donor groupings can have substantial implications for healthcare logistics and resource allocation. These insights pave the way for further research into the optimization of clustering techniques in dynamic datasets and their practical applications in medical and other domains. |
Keywords | K-Means clustering, Hierarchical Clustering, DBSCAN, Blood Donation |
Field | Engineering |
Published In | Volume 6, Issue 4, July-August 2024 |
Published On | 2024-08-30 |
DOI | https://doi.org/10.36948/ijfmr.2024.v06i04.26755 |
Short DOI | https://doi.org/gt8gvg |
Share this

E-ISSN 2582-2160

CrossRef DOI is assigned to each research paper published in our journal.
IJFMR DOI prefix is
10.36948/ijfmr
Downloads
All research papers published on this website are licensed under Creative Commons Attribution-ShareAlike 4.0 International License, and all rights belong to their respective authors/researchers.
