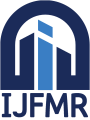
International Journal For Multidisciplinary Research
E-ISSN: 2582-2160
•
Impact Factor: 9.24
A Widely Indexed Open Access Peer Reviewed Multidisciplinary Bi-monthly Scholarly International Journal
Home
Research Paper
Submit Research Paper
Publication Guidelines
Publication Charges
Upload Documents
Track Status / Pay Fees / Download Publication Certi.
Editors & Reviewers
View All
Join as a Reviewer
Get Membership Certificate
Current Issue
Publication Archive
Conference
Publishing Conf. with IJFMR
Upcoming Conference(s) ↓
WSMCDD-2025
GSMCDD-2025
AIMAR-2025
Conferences Published ↓
ICCE (2025)
RBS:RH-COVID-19 (2023)
ICMRS'23
PIPRDA-2023
Contact Us
Plagiarism is checked by the leading plagiarism checker
Call for Paper
Volume 7 Issue 4
July-August 2025
Indexing Partners






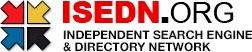

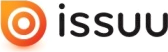


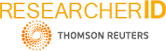
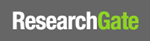
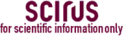
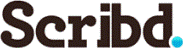

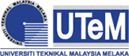
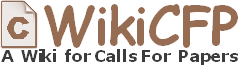
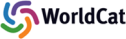
Comparison of Various Variant Prediction Algorithms and Conservation Scores by Machine Learning Approaches
Author(s) | Sombuddha Roy Bhowmick |
---|---|
Country | India |
Abstract | Machine learning methods are widely used for prediction of the pathogenicity and deleteriousness of the different types of single nucleotide variants (SNVs). This study encompasses a preliminary approach towards the application of different types of machine learning algorithms on the dbNSFPv4.7 (database of Nonsynonymous SNPs Functional Predictions) database to compare the various prediction algorithms and conservation scores to determine which one performs the best. This paves the way for selecting the best prediction algorithm or the combination of the better prediction algorithms in annotation of the variants and classifying them as pathogenic or likely pathogenic, benign or likely benign. The different learning approaches were compared based on the metrices like, accuracy, recall, precision and F1 score. Among the different variant prediction algorithms and conservation scores available in the dbNSFP database, BayesDel AF was the best perfoming followed by BayesDel noAF, MetaRNN, MCAP and MutPred. bStatistic, fitCons, fathmm XF, MutationTaster and phastCons17way primate performed the worst among 31 prediction algorithms and 9 conservation scores selected for this study. The best performing features or instances can be prioritized over others while selecting the functional prediction algorithms, which are used to determine the driver mutations or the pathogenic variants from omics datasets. |
Keywords | Machine Learning, Prediction Algorithms, Conservation Scores, Single Nucleotide Variants (SNVs), dbNSFP |
Field | Biology |
Published In | Volume 6, Issue 5, September-October 2024 |
Published On | 2024-09-05 |
DOI | https://doi.org/10.36948/ijfmr.2024.v06i05.27138 |
Short DOI | https://doi.org/gwfgrm |
Share this

E-ISSN 2582-2160

CrossRef DOI is assigned to each research paper published in our journal.
IJFMR DOI prefix is
10.36948/ijfmr
Downloads
All research papers published on this website are licensed under Creative Commons Attribution-ShareAlike 4.0 International License, and all rights belong to their respective authors/researchers.
