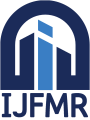
International Journal For Multidisciplinary Research
E-ISSN: 2582-2160
•
Impact Factor: 9.24
A Widely Indexed Open Access Peer Reviewed Multidisciplinary Bi-monthly Scholarly International Journal
Home
Research Paper
Submit Research Paper
Publication Guidelines
Publication Charges
Upload Documents
Track Status / Pay Fees / Download Publication Certi.
Editors & Reviewers
View All
Join as a Reviewer
Reviewer Referral Program
Get Membership Certificate
Current Issue
Publication Archive
Conference
Publishing Conf. with IJFMR
Upcoming Conference(s) ↓
WSMCDD-2025
GSMCDD-2025
Conferences Published ↓
RBS:RH-COVID-19 (2023)
ICMRS'23
PIPRDA-2023
Contact Us
Plagiarism is checked by the leading plagiarism checker
Call for Paper
Volume 6 Issue 6
November-December 2024
Indexing Partners






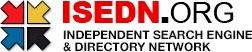

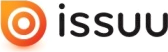


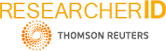
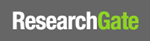
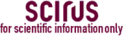
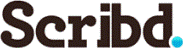

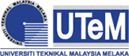
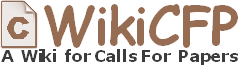
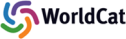
Effective Detection and Mitigation of Browser Hijacking Using Deep Learning Models
Author(s) | Sambhav Patil, Chandra Sekhar Dash |
---|---|
Country | India |
Abstract | Deep learning algorithms have been employed in the detection and prevention of browser hijacking and this research paper seeks to compare the efficiency of the different deep learning algorithms. Browser hijacking is a serious form of cybercrime that affects major internet browsers, and entails the changing of a browser’s settings or behavior without the consent of a user, and generally results in detrimental effects on the privacy and security of a user. To address this challenge, we evaluate five deep learning models: Some of the major types of neural networks are Convolutional Neural Networks (CNNs), Recurrent Neural Networks (RNNs), Long Short-Term Memory Networks (LSTMs), Autoencoders and Graph Neural Networks (GNNs). In each model, the training and testing data set included the network traffic records, browser activity recordings, screen shots and the system logs. Specifically, for the evaluation of these models the following parameters of detection: accuracy, false positive rate, false negative rate, precision, recall, the F1 factor, the AUC score. I found CNN model to have the highest detection accuracy of 93. 5% and the highest AUC score of 0. 96 showing that CNN was more effective in detecting the hijacking and the benign activities. Other represented algorithms, LSTM and GNN also showed a high level of accuracy with values 91. 2% and 90. 3% accordingly. The accuracy of the RNN model was satisfactory yet its false positive as well as false negative numbers were lower. The used Autoencoder model was most suitable for data anomaly detection but it presented the lowest accuracy and the highest error. The results point out the merits and demerits of each algorithm and indicate that integration of models can boost total identification capacities. All in all, it is possible to underline that this research provides a significant contribution into investigating the applicability of deep learning methodologies for mitigating browser hijacking and enhances the existing knowledge and concepts in the field of cybersecurity. |
Keywords | browser hijacking, deep learning, Convolutional Neural Networks (CNNs), Recurrent Neural Networks (RNNs), Long Short-Term Memory Networks (LSTMs), Autoencoders |
Field | Computer > Network / Security |
Published In | Volume 6, Issue 5, September-October 2024 |
Published On | 2024-09-09 |
Cite This | Effective Detection and Mitigation of Browser Hijacking Using Deep Learning Models - Sambhav Patil, Chandra Sekhar Dash - IJFMR Volume 6, Issue 5, September-October 2024. DOI 10.36948/ijfmr.2024.v06i05.27273 |
DOI | https://doi.org/10.36948/ijfmr.2024.v06i05.27273 |
Short DOI | https://doi.org/gwfgkj |
Share this

E-ISSN 2582-2160

CrossRef DOI is assigned to each research paper published in our journal.
IJFMR DOI prefix is
10.36948/ijfmr
Downloads
All research papers published on this website are licensed under Creative Commons Attribution-ShareAlike 4.0 International License, and all rights belong to their respective authors/researchers.
