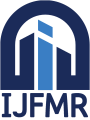
International Journal For Multidisciplinary Research
E-ISSN: 2582-2160
•
Impact Factor: 9.24
A Widely Indexed Open Access Peer Reviewed Multidisciplinary Bi-monthly Scholarly International Journal
Home
Research Paper
Submit Research Paper
Publication Guidelines
Publication Charges
Upload Documents
Track Status / Pay Fees / Download Publication Certi.
Editors & Reviewers
View All
Join as a Reviewer
Get Membership Certificate
Current Issue
Publication Archive
Conference
Publishing Conf. with IJFMR
Upcoming Conference(s) ↓
WSMCDD-2025
GSMCDD-2025
Conferences Published ↓
RBS:RH-COVID-19 (2023)
ICMRS'23
PIPRDA-2023
Contact Us
Plagiarism is checked by the leading plagiarism checker
Call for Paper
Volume 7 Issue 2
March-April 2025
Indexing Partners






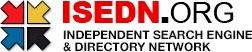

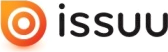


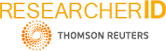
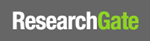
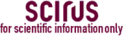
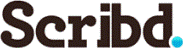

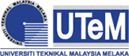
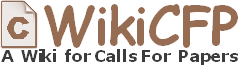
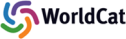
Quantum Machine Learning: Leveraging Quantum Computing for Enhanced Learning Algorithms
Author(s) | Sonia Rani, Ravinder Kaur, Chitra Desai, R.P.Ambilwade |
---|---|
Country | India |
Abstract | The paper "Quantum Machine Learning: Leveraging Quantum Computing for Enhanced Learning Algorithms" explores the integration of quantum computing principles into classical machine learning techniques, aiming to address limitations such as scalability and computational inefficiency. It presents the foundational concepts of quantum computing, including superposition and entanglement, and their application in accelerating machine learning processes. The study emphasizes the potential for quantum algorithms to significantly improve the performance of machine learning tasks by processing large datasets more efficiently and exploring larger hypothesis spaces. Key quantum machine learning algorithms discussed include Quantum Support Vector Machines (QSVM), Quantum Principal Component Analysis (QPCA), and Quantum Neural Networks (QNN), each of which leverages quantum mechanics to overcome the computational barriers faced by classical algorithms. The Quantum Approximate Optimization Algorithm (QAOA) is also highlighted for its ability to optimize machine learning models more effectively. While the theoretical benefits of Quantum Machine Learning (QML) are promising, the practical application of these techniques is currently limited by the constraints of existing quantum hardware. This research contributes to the emerging field of QML by examining its potential advantages and future implications in addressing complex data processing challenges. |
Keywords | Keywords - Quantum Machine Learning (QML), Quantum Computing Algorithms, Quantum Support Vector Machines (QSVM), Quantum Neural Networks (QNN), Quantum Approximate Optimization Algorithm (QAOA). |
Field | Engineering |
Published In | Volume 6, Issue 5, September-October 2024 |
Published On | 2024-09-13 |
Cite This | Quantum Machine Learning: Leveraging Quantum Computing for Enhanced Learning Algorithms - Sonia Rani, Ravinder Kaur, Chitra Desai, R.P.Ambilwade - IJFMR Volume 6, Issue 5, September-October 2024. DOI 10.36948/ijfmr.2024.v06i05.27450 |
DOI | https://doi.org/10.36948/ijfmr.2024.v06i05.27450 |
Short DOI | https://doi.org/gzwp3s |
Share this

E-ISSN 2582-2160

CrossRef DOI is assigned to each research paper published in our journal.
IJFMR DOI prefix is
10.36948/ijfmr
Downloads
All research papers published on this website are licensed under Creative Commons Attribution-ShareAlike 4.0 International License, and all rights belong to their respective authors/researchers.
