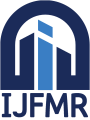
International Journal For Multidisciplinary Research
E-ISSN: 2582-2160
•
Impact Factor: 9.24
A Widely Indexed Open Access Peer Reviewed Multidisciplinary Bi-monthly Scholarly International Journal
Home
Research Paper
Submit Research Paper
Publication Guidelines
Publication Charges
Upload Documents
Track Status / Pay Fees / Download Publication Certi.
Editors & Reviewers
View All
Join as a Reviewer
Get Membership Certificate
Current Issue
Publication Archive
Conference
Publishing Conf. with IJFMR
Upcoming Conference(s) ↓
WSMCDD-2025
GSMCDD-2025
Conferences Published ↓
RBS:RH-COVID-19 (2023)
ICMRS'23
PIPRDA-2023
Contact Us
Plagiarism is checked by the leading plagiarism checker
Call for Paper
Volume 7 Issue 2
March-April 2025
Indexing Partners






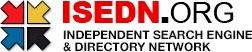

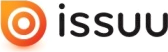


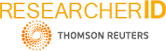
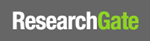
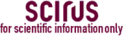
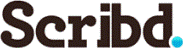

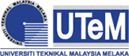
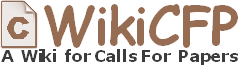
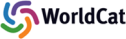
Design and Analysis on Detection and Prediction Model Based on Explainable Artificial Intelligence for Alzheimer’s Disease
Author(s) | Mrs. Rani. Burugu, Dr. Dr. S.K. Mohana Sundar, Mrs.Vandana Nandigama, Nagamani Naama, Mrs. Rasmi Singh |
---|---|
Country | India |
Abstract | The Alzheimer's disease (AD) that causes dementia in most people. Its diagnostic and progression-detecting capabilities have been the subject of substantial study. Research findings rarely impact clinical practice, though, and this is due to the following factors: (1) neuroimaging is the primary area of study; (2) the separation of diagnosis and progression detection is common practice; and (3) optimization of complicated machine learning models' performance, not their explainability, is the main emphasis of current studies. This complicates matters and makes it tougher for physicians to have faith in these models. A reliable and understandable model for monitoring the progression of Alzheimer's disease is meticulously constructed in this study. Practitioners are able to give educated recommendations with thorough explanations when they employ this assessment method. The ADNI real-world dataset contains information from 1048 participants, and the model uses eleven different kinds of imaging data from that dataset. Out of the total population, 294 have normal cognitive capacities, 254 have stable moderate cognitive impairment (MCI), 232 have MCI that is getting worse, and 268 have severe symptoms of Alzheimer's disease (AD). Among the several approaches offered by various authors in this book is a two-layer model that makes use of random forest (RF) as a classification technique. To identify early signs of Alzheimer's disease, the model's initial layer use multi-class classification. Layer two of the model uses binary classification to assess the patient's chance of getting Alzheimer's disease (AD) within three years after a moderate cognitive impairment (MCI) diagnosis. A wide variety of biological and clinical characteristics are used to pick essential markers, which improves the model's accuracy. We describe the global and instance-by-instance explainability of the RF classifier's layers using the SHapley Additive exPlanations (SHAP) feature attribution paradigm |
Field | Computer Applications |
Published In | Volume 6, Issue 5, September-October 2024 |
Published On | 2024-09-21 |
DOI | https://doi.org/10.36948/ijfmr.2024.v06i05.27728 |
Short DOI | https://doi.org/g4qmjm |
Share this

E-ISSN 2582-2160

CrossRef DOI is assigned to each research paper published in our journal.
IJFMR DOI prefix is
10.36948/ijfmr
Downloads
All research papers published on this website are licensed under Creative Commons Attribution-ShareAlike 4.0 International License, and all rights belong to their respective authors/researchers.
