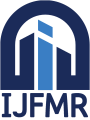
International Journal For Multidisciplinary Research
E-ISSN: 2582-2160
•
Impact Factor: 9.24
A Widely Indexed Open Access Peer Reviewed Multidisciplinary Bi-monthly Scholarly International Journal
Home
Research Paper
Submit Research Paper
Publication Guidelines
Publication Charges
Upload Documents
Track Status / Pay Fees / Download Publication Certi.
Editors & Reviewers
View All
Join as a Reviewer
Get Membership Certificate
Current Issue
Publication Archive
Conference
Publishing Conf. with IJFMR
Upcoming Conference(s) ↓
WSMCDD-2025
GSMCDD-2025
Conferences Published ↓
RBS:RH-COVID-19 (2023)
ICMRS'23
PIPRDA-2023
Contact Us
Plagiarism is checked by the leading plagiarism checker
Call for Paper
Volume 7 Issue 2
March-April 2025
Indexing Partners






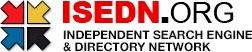

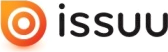


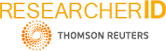
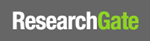
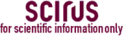
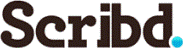

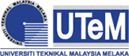
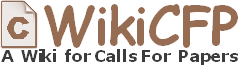
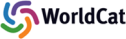
Federated Learning Frameworks for Secure and Decentralized Authentication
Author(s) | Merlin Balamurugan |
---|---|
Country | United States |
Abstract | Federated Learning (FL) is a cutting-edge machine learning approach that enables multiple or edge devices to train models collaboratively without sharing sensitive data. This approach not only ensures the privacy and security of data by keeping it localized but also promotes the collective improvement of machine learning models across various participants. A systematic literature review explored integrating Blockchain technology with federated learning. Blockchain's potential to address existing security and privacy vulnerabilities in traditional federated learning systems is analyzed in depth. One of the key benefits of combining Blockchain with FL is enhanced protection against potential attacks, such as data tampering or unauthorized access. The study also examines how Blockchain-based federated learning systems can offer better records and rewards management, contributing to fairer and more transparent systems. In addition, Blockchain's role in improving verification and accountability within federated learning frameworks has been critically evaluated. By integrating Blockchain, federated learning can achieve higher levels of trust and security in collaborative machine-learning processes. The latest research highlights innovative Blockchain-based methods that tackle these challenges, ensuring robust privacy and security measures. Overall, this approach represents a significant advancement in distributed machine learning, aligning with contemporary needs for data protection and collaborative efficiency. |
Keywords | Federated Learning, Distributed machine learning, Blockchain, Smart contract, Privacy and Security |
Field | Computer > Artificial Intelligence / Simulation / Virtual Reality |
Published In | Volume 6, Issue 5, September-October 2024 |
Published On | 2024-09-26 |
DOI | https://doi.org/10.36948/ijfmr.2024.v06i05.27901 |
Short DOI | https://doi.org/g59zvx |
Share this

E-ISSN 2582-2160

CrossRef DOI is assigned to each research paper published in our journal.
IJFMR DOI prefix is
10.36948/ijfmr
Downloads
All research papers published on this website are licensed under Creative Commons Attribution-ShareAlike 4.0 International License, and all rights belong to their respective authors/researchers.
