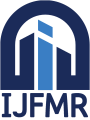
International Journal For Multidisciplinary Research
E-ISSN: 2582-2160
•
Impact Factor: 9.24
A Widely Indexed Open Access Peer Reviewed Multidisciplinary Bi-monthly Scholarly International Journal
Home
Research Paper
Submit Research Paper
Publication Guidelines
Publication Charges
Upload Documents
Track Status / Pay Fees / Download Publication Certi.
Editors & Reviewers
View All
Join as a Reviewer
Reviewer Referral Program
Get Membership Certificate
Current Issue
Publication Archive
Conference
Publishing Conf. with IJFMR
Upcoming Conference(s) ↓
WSMCDD-2025
GSMCDD-2025
Conferences Published ↓
RBS:RH-COVID-19 (2023)
ICMRS'23
PIPRDA-2023
Contact Us
Plagiarism is checked by the leading plagiarism checker
Call for Paper
Volume 6 Issue 6
November-December 2024
Indexing Partners






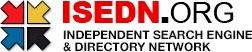

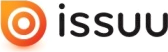


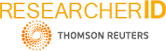
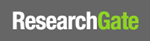
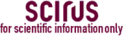
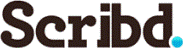

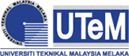
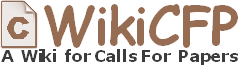
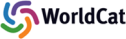
Deep Reinforcement Learning for Autonomous Driving Systems
Author(s) | Sanskar Jadhav, Vedant Sonwalkar, Shweta Shewale, Pranav Shitole, Samarth Bhujadi |
---|---|
Country | India |
Abstract | Autonomous driving systems (ADS) are poised to revolutionize the future of transportation, promising increased safety, efficiency, and convenience. Deep Reinforcement Learning (DRL) has emerged as a powerful approach to solving complex decision-making tasks in dynamic environments, making it a promising candidate for the development of intelligent autonomous vehicles. This paper explores the application of DRL techniques in autonomous driving, focusing on the integration of perception, planning, and control. We review state-of-the-art DRL algorithms, including Deep Q-Networks (DQN), Proximal Policy Optimization (PPO), and Soft Actor-Critic (SAC), and examine their roles in enabling end-to-end learning for driving policies. Furthermore, we discuss the challenges inherent in deploying DRL in real-world autonomous driving scenarios, including sample inefficiency, safety constraints, and the sim-to-real gap. Finally, the paper presents case studies and experimental results that highlight the potential of DRL to improve autonomous vehicle performance in complex environments, while identifying future research directions to address open problems in the field. |
Keywords | Deep Reinforcement Learning (DRL), Autonomous Driving Systems (ADS), Deep Q-Networks (DQN), Proximal Policy Optimization (PPO), Soft Actor-Critic (SAC), End-to-End Learning, Sim-to-Real Transfer, Perception and Control, Safe Autonomous Driving, Policy Learning. |
Field | Engineering |
Published In | Volume 6, Issue 5, September-October 2024 |
Published On | 2024-10-08 |
Cite This | Deep Reinforcement Learning for Autonomous Driving Systems - Sanskar Jadhav, Vedant Sonwalkar, Shweta Shewale, Pranav Shitole, Samarth Bhujadi - IJFMR Volume 6, Issue 5, September-October 2024. DOI 10.36948/ijfmr.2024.v06i05.28518 |
DOI | https://doi.org/10.36948/ijfmr.2024.v06i05.28518 |
Short DOI | https://doi.org/g7943h |
Share this

E-ISSN 2582-2160

CrossRef DOI is assigned to each research paper published in our journal.
IJFMR DOI prefix is
10.36948/ijfmr
Downloads
All research papers published on this website are licensed under Creative Commons Attribution-ShareAlike 4.0 International License, and all rights belong to their respective authors/researchers.
